Deck 16: Regression Models for Nonlinear Relationships
Question
Question
Question
Question
Question
Question
Question
Question
Question
Question
Question
Question
Question
Question
Question
Question
Question
Question
Question
Question
Question
Question
Question
Question
Question
Question
Question
Question
Question
Question
Question
Question
Question
Question
Question
Question
Question
Question
Question
Question
Question
Question
Question
Question
Question
Question
Question
Question
Question
Question
Question
Question
Question
Question
Question
Question
Question
Question
Question
Question
Question
Question
Question
Question
Question
Question
Question
Question
Question
Question
Question
Question
Question
Question
Question
Question
Question
Question
Question
Question
Unlock Deck
Sign up to unlock the cards in this deck!
Unlock Deck
Unlock Deck
1/118
Play
Full screen (f)
Deck 16: Regression Models for Nonlinear Relationships
1
The fit of the regression equations
= b0 + b1x + b2x2 and
= b0 + b1x + b2x2 + b3x3 can be compared using the coefficient of determination R2.


False
2
For the log-log model ln(y) = β0 + β1ln(x) + ε, β1 is the approximate percent change in E(y) when x increases by 1%.
True
3
For the exponential model ln(y) = β0 + β1x + ε, β1 × 100% is the approximate percentage change in E(y) when x increases by 1%.
False
4
The cubic regression model allows for ________ change(s) in slope.
Unlock Deck
Unlock for access to all 118 flashcards in this deck.
Unlock Deck
k this deck
5
When not all variables are transformed with logarithms, the models are called ________ models.
Unlock Deck
Unlock for access to all 118 flashcards in this deck.
Unlock Deck
k this deck
6
The fit of the models y = β0 + β1x + ε and y = β0 + β1ln(x) + ε can be compared using the coefficient of determination R2.
Unlock Deck
Unlock for access to all 118 flashcards in this deck.
Unlock Deck
k this deck
7
The regression model ln(y) = β0 + β1x + ε is called a logarithmic model.
Unlock Deck
Unlock for access to all 118 flashcards in this deck.
Unlock Deck
k this deck
8
For the quadratic regression model
= b0 + b1x + b2x2, b1 can be interpreted as the change in the predicted value of y when x increases by 1 unit.

Unlock Deck
Unlock for access to all 118 flashcards in this deck.
Unlock Deck
k this deck
9
The log-log regression model is ________ in the variables.
Unlock Deck
Unlock for access to all 118 flashcards in this deck.
Unlock Deck
k this deck
10
For the logarithmic model y = β0 + β1ln(x) + ε, β1 × 100% is the approximate percentage change in E(y) when x increases by 1%.
Unlock Deck
Unlock for access to all 118 flashcards in this deck.
Unlock Deck
k this deck
11
The quadratic regression model
= b0 + b1x + b2x2 reaches a maximum when b2 < 0.

Unlock Deck
Unlock for access to all 118 flashcards in this deck.
Unlock Deck
k this deck
12
The curve representing the regression equation
= b0 + b1x + b2x2 has a U-shape if b2 < 0.

Unlock Deck
Unlock for access to all 118 flashcards in this deck.
Unlock Deck
k this deck
13
The quadratic regression model
= b0 + b1x + b2x2 allows for one sign change in the slope capturing the influence of x on y.

Unlock Deck
Unlock for access to all 118 flashcards in this deck.
Unlock Deck
k this deck
14
The regression model ln(y) = β0 + β1x + ε is called an exponential model.
Unlock Deck
Unlock for access to all 118 flashcards in this deck.
Unlock Deck
k this deck
15
The regression model ln(y) = β0 + β1ln(x) + ε is called a log-log model.
Unlock Deck
Unlock for access to all 118 flashcards in this deck.
Unlock Deck
k this deck
16
The cubic regression model
= b0 + b1x + b2x2 + b3x3 allows for one sign change in the slope capturing the influence of x on y.

Unlock Deck
Unlock for access to all 118 flashcards in this deck.
Unlock Deck
k this deck
17
The quadratic regression model allows for one change in ________.
Unlock Deck
Unlock for access to all 118 flashcards in this deck.
Unlock Deck
k this deck
18
A quadratic regression model is a polynomial regression model of order 2.
Unlock Deck
Unlock for access to all 118 flashcards in this deck.
Unlock Deck
k this deck
19
A cubic regression model is a polynomial regression model of order 2.
Unlock Deck
Unlock for access to all 118 flashcards in this deck.
Unlock Deck
k this deck
20
The log-log and the ________ models can have similar shapes.
Unlock Deck
Unlock for access to all 118 flashcards in this deck.
Unlock Deck
k this deck
21
For the quadratic regression equation
= b0 + b1x + b2x2, the predicted y achieves its optimum (maximum or minimum) when x is ________.
A) -2b2/b1
B) -b1/2b2
C) b1/2b2
D) 2b1/b2

A) -2b2/b1
B) -b1/2b2
C) b1/2b2
D) 2b1/b2
Unlock Deck
Unlock for access to all 118 flashcards in this deck.
Unlock Deck
k this deck
22
Which of the following regression models is a first-order polynomial?
A) y = β0 + ε
B) y = β0 + β1x + ε
C) y = β0 + β1x +β2x2+ ε
D) y = β0 + β1x + β2x2 + β3x3 + ε
A) y = β0 + ε
B) y = β0 + β1x + ε
C) y = β0 + β1x +β2x2+ ε
D) y = β0 + β1x + β2x2 + β3x3 + ε
Unlock Deck
Unlock for access to all 118 flashcards in this deck.
Unlock Deck
k this deck
23
The following scatterplot shows productivity and number hired workers with a fitted quadratic regression model.
Assuming that the number of hired workers must be integer, how many workers should be hired to achieve the highest productivity according to the model?
A) 26
B) 28
C) 30
D) 32
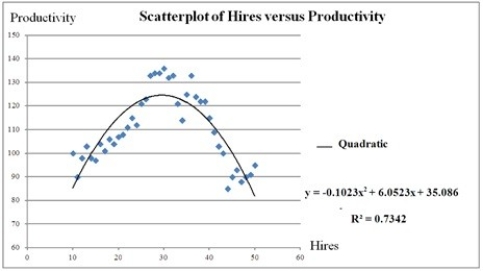
A) 26
B) 28
C) 30
D) 32
Unlock Deck
Unlock for access to all 118 flashcards in this deck.
Unlock Deck
k this deck
24
To compute the coefficient of determination R2 we have to use Excel's ________ function first to compute the correlation between y and
.

Unlock Deck
Unlock for access to all 118 flashcards in this deck.
Unlock Deck
k this deck
25
For the quadratic equation
= b0 + b1x + b2x2, which of the following expressions must be zero in order to minimize or maximize the predicted y?
A) b1 + 2b2x
B) 2b1 + b2x
C) -b1/2b2
D) -b2/2b2

A) b1 + 2b2x
B) 2b1 + b2x
C) -b1/2b2
D) -b2/2b2
Unlock Deck
Unlock for access to all 118 flashcards in this deck.
Unlock Deck
k this deck
26
The following scatterplot shows productivity and number hired workers with a fitted quadratic regression model.
Assuming that the number of hired workers must be an integer, what is the maximum productivity predicted by the model?
A) 29.58
B) 30.00
C) 124.603
D) 124.585
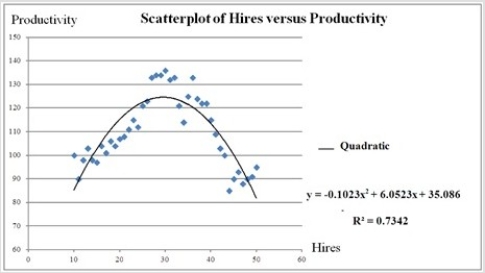
A) 29.58
B) 30.00
C) 124.603
D) 124.585
Unlock Deck
Unlock for access to all 118 flashcards in this deck.
Unlock Deck
k this deck
27
The following scatterplot shows productivity and number hired workers with a fitted quadratic regression model.
The quadratic regression model is ________.
A)
= 35.086 + 6.0523Hires - 0.1023Hires2
B)
= 6.0523 + 35.086Hires - 0.1023Hires2
C)
= 6.0523 − 35.086Hires + 0.1023Hires2
D)
= −0.1023 + 6.0523Hires + 35.086Hires2
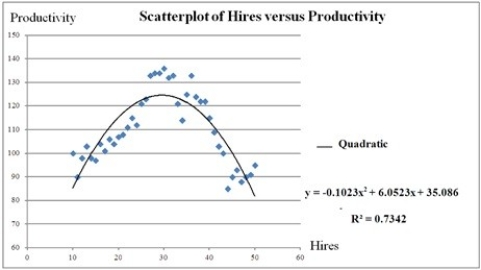
A)

B)

C)

D)

Unlock Deck
Unlock for access to all 118 flashcards in this deck.
Unlock Deck
k this deck
28
How many coefficients need to be estimated in the quadratic regression model?
A) 4
B) 3
C) 2
D) 1
A) 4
B) 3
C) 2
D) 1
Unlock Deck
Unlock for access to all 118 flashcards in this deck.
Unlock Deck
k this deck
29
For a quadratic regression model , it is important to evaluate the estimated ________ effect of the explanatory variable x on the predicted value of the response variable
.

Unlock Deck
Unlock for access to all 118 flashcards in this deck.
Unlock Deck
k this deck
30
What is the effect of b2 < 0 in the case of the quadratic equation
= b0 + b1x + b2x2?
A) The curve is U-shaped.
B) The curve is inverted U-shaped.
C) The curve is a straight line.
D) The curve is not a parabola.

A) The curve is U-shaped.
B) The curve is inverted U-shaped.
C) The curve is a straight line.
D) The curve is not a parabola.
Unlock Deck
Unlock for access to all 118 flashcards in this deck.
Unlock Deck
k this deck
31
For the quadratic regression equation
= b0 + b1x + b2x2, the optimum (maximum or minimum) value of
is ________.
A) -b1/2b2
B) b1/2b2
C) b0 -
D) b0 -


A) -b1/2b2
B) b1/2b2
C) b0 -

D) b0 -

Unlock Deck
Unlock for access to all 118 flashcards in this deck.
Unlock Deck
k this deck
32
The following scatterplot shows productivity and number hired workers with a fitted quadratic regression model.
Assuming that the values of Hires can be nonintegers, what is the maximum value of Productivity predicted by the model?
A) 29.58
B) 124.603
C) 35.086
D) 127.50
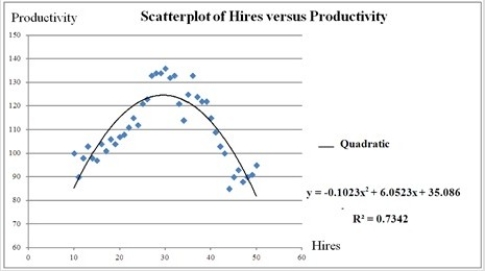
A) 29.58
B) 124.603
C) 35.086
D) 127.50
Unlock Deck
Unlock for access to all 118 flashcards in this deck.
Unlock Deck
k this deck
33
To compute the coefficient of determination R2 we have to use R's ________ function first to compute the correlation between y and
.

Unlock Deck
Unlock for access to all 118 flashcards in this deck.
Unlock Deck
k this deck
34
The following scatterplot shows productivity and number hired workers with a fitted quadratic regression model.
Which of the following is the predicted productivity when 32 workers are hired?
A) 124.00
B) 122.46
C) 121.60
D) 113.50
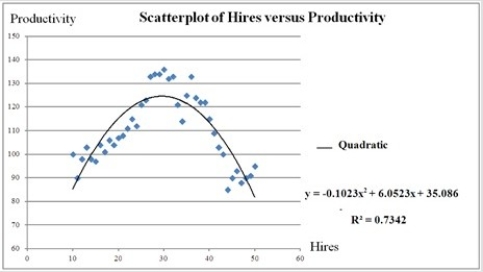
A) 124.00
B) 122.46
C) 121.60
D) 113.50
Unlock Deck
Unlock for access to all 118 flashcards in this deck.
Unlock Deck
k this deck
35
Although allowing for nonlinear trends, polynomials are still linear in the ________.
A) response
B) explanatory variables
C) coefficients
D) none of these options
A) response
B) explanatory variables
C) coefficients
D) none of these options
Unlock Deck
Unlock for access to all 118 flashcards in this deck.
Unlock Deck
k this deck
36
The logarithmic model is especially attractive when only the ________ variable is better captured in percentages.
Unlock Deck
Unlock for access to all 118 flashcards in this deck.
Unlock Deck
k this deck
37
An inverted U-shaped curve is also known as ________.
A) concave
B) convex
C) opaque
D) hyperbola
A) concave
B) convex
C) opaque
D) hyperbola
Unlock Deck
Unlock for access to all 118 flashcards in this deck.
Unlock Deck
k this deck
38
Which of the following is a quadratic regression equation?
A) y = β0 + ε
B) y = β0 + β1x + ε
C) y = β0 + β1x +β2x2+ ε
D) y = β0 + β1x + β2x2 + β3x3 + ε
A) y = β0 + ε
B) y = β0 + β1x + ε
C) y = β0 + β1x +β2x2+ ε
D) y = β0 + β1x + β2x2 + β3x3 + ε
Unlock Deck
Unlock for access to all 118 flashcards in this deck.
Unlock Deck
k this deck
39
The following scatterplot shows productivity and number hired workers with a fitted quadratic regression model.
For which value of Hires is the predicted Productivity maximized? Note: Do not round to the nearest integer.
A) 29.58
B) 124.60
C) 35.086
D) 27.34
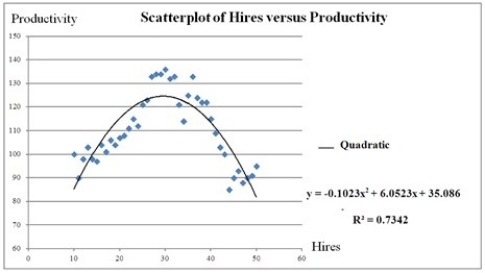
A) 29.58
B) 124.60
C) 35.086
D) 27.34
Unlock Deck
Unlock for access to all 118 flashcards in this deck.
Unlock Deck
k this deck
40
The following scatterplot shows productivity and number hired workers with a fitted quadratic regression model.
What is percentage of the variation in productivity is explained by the quadratic regression model?
A) 85.69%
B) 0.7342%
C) 90.54%
D) 73.42%
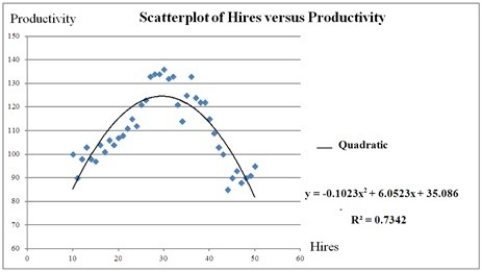
A) 85.69%
B) 0.7342%
C) 90.54%
D) 73.42%
Unlock Deck
Unlock for access to all 118 flashcards in this deck.
Unlock Deck
k this deck
41
A model in which both the response variable and the explanatory variable have been log transformed is called a(n) ________.
A) exponential model
B) logarithmic model
C) linear model
D) log-log model
A) exponential model
B) logarithmic model
C) linear model
D) log-log model
Unlock Deck
Unlock for access to all 118 flashcards in this deck.
Unlock Deck
k this deck
42
Typically, the sales volume declines with an increase of a product price. It has been observed, however, that for some luxury goods the sales volume may increase when the price increases. The following scatterplot illustrates this rather unusual relationship.
For which of the following prices do sales predicted by the quadratic regression equation reach their minimum?
A) 106.33
B) 1157.16
C) 100.41
D) 1166.64
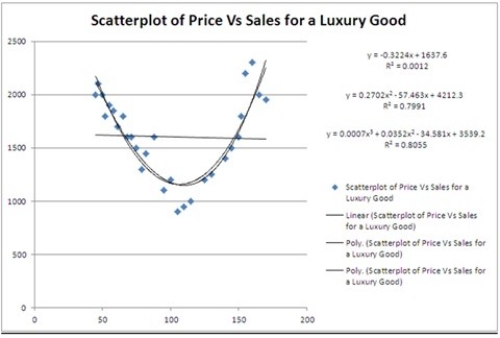
A) 106.33
B) 1157.16
C) 100.41
D) 1166.64
Unlock Deck
Unlock for access to all 118 flashcards in this deck.
Unlock Deck
k this deck
43
For the exponential model ln(y) = β0 + β1x + ε, if x increases by one unit, then E(y) changes by approximately
A) β1 × 100%
B) β1 × 100 units
C) β1%
D) β1 units
A) β1 × 100%
B) β1 × 100 units
C) β1%
D) β1 units
Unlock Deck
Unlock for access to all 118 flashcards in this deck.
Unlock Deck
k this deck
44
What R function is used to fit a quadratic regression model?
A) lm
B) predict
C) summary
D) cor
A) lm
B) predict
C) summary
D) cor
Unlock Deck
Unlock for access to all 118 flashcards in this deck.
Unlock Deck
k this deck
45
Typically, the sales volume declines with an increase of a product price. It has been observed, however, that for some luxury goods the sales volume may increase when the price increases. The following scatterplot illustrates this rather unusual relationship.
For the considered range of the price, the relationship between Price and Sales should be described by a ________.
A) concave function
B) hyperbola
C) convex function
D) linear function
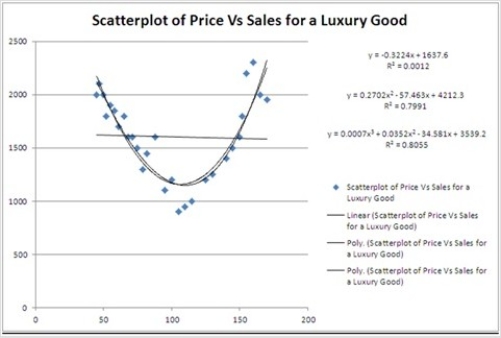
A) concave function
B) hyperbola
C) convex function
D) linear function
Unlock Deck
Unlock for access to all 118 flashcards in this deck.
Unlock Deck
k this deck
46
Typically, the sales volume declines with an increase of a product price. It has been observed, however, that for some luxury goods the sales volume may increase when the price increases. The following scatterplot illustrates this rather unusual relationship.
For which of the following two prices are the sales predicted by the quadratic regression equation equal 1700 units?
A) 60.51 and 150.15
B) 61.51 and 151.15
C) 62.51 and 152.15
D) 63.51 and 153.15
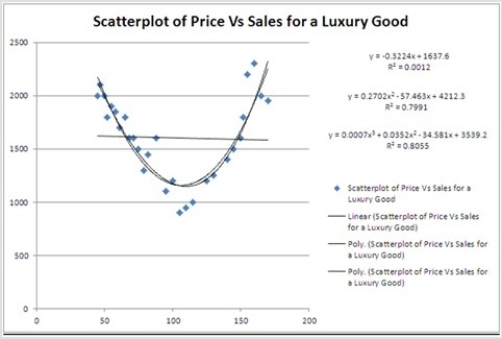
A) 60.51 and 150.15
B) 61.51 and 151.15
C) 62.51 and 152.15
D) 63.51 and 153.15
Unlock Deck
Unlock for access to all 118 flashcards in this deck.
Unlock Deck
k this deck
47
The coefficient of determination R2 cannot be used to compare the linear and quadratic models, because
A) the quadratic model has one parameter more to estimate.
B) the quadratic model has two parameters more to estimate.
C) the quadratic model always has a lower R2.
D) R2 is not defined for the quadratic model.
A) the quadratic model has one parameter more to estimate.
B) the quadratic model has two parameters more to estimate.
C) the quadratic model always has a lower R2.
D) R2 is not defined for the quadratic model.
Unlock Deck
Unlock for access to all 118 flashcards in this deck.
Unlock Deck
k this deck
48
What does a positive value for price elasticity indicate if y represents the quantity demanded of a particular good and x is its unit price in a log-log regression model?
A) As price increases, the expected sales decreases.
B) As price decreases, the expected sales increases.
C) As price increases, the expected sales increases.
D) As price decreases, the expected sales remain the same.
A) As price increases, the expected sales decreases.
B) As price decreases, the expected sales increases.
C) As price increases, the expected sales increases.
D) As price decreases, the expected sales remain the same.
Unlock Deck
Unlock for access to all 118 flashcards in this deck.
Unlock Deck
k this deck
49
For the log-log model ln(y) = β0 + β1ln(x) + ε, the predicted value of y is computed as ________.
A)
= b0 + b1x
B)
= exp(b0 + b1ln(x) +
/2)
C)
= b0 + b1ln(x)
D)
= exp(b0 + b1x +
/2)
A)

B)


C)

D)


Unlock Deck
Unlock for access to all 118 flashcards in this deck.
Unlock Deck
k this deck
50
For the logarithmic model ln(y) = β0 + β1ln(x) + ε, the predicted value of y is computed as ________.
A)
= exp(b0 + b1ln(x) +
/2)
B)
= b0 + b1ln(x)
C)
= b0 + b1x
D)
= exp(b0 + b1x +
/2)
A)


B)

C)

D)


Unlock Deck
Unlock for access to all 118 flashcards in this deck.
Unlock Deck
k this deck
51
A model with one explanatory variable that has been log transformed is called a(n) ________.
A) log-log model
B) logarithmic model
C) exponential model
D) linear model
A) log-log model
B) logarithmic model
C) exponential model
D) linear model
Unlock Deck
Unlock for access to all 118 flashcards in this deck.
Unlock Deck
k this deck
52
Typically, the sales volume declines with an increase of a product price. It has been observed, however, that for some luxury goods the sales volume may increase when the price increases. The following scatterplot illustrates this rather unusual relationship.
Using the cubic regression equation, predict the sales if the luxury good is priced at $100.
A) 1171.85
B) 1133.10
C) 1106.61
D) 1092.91
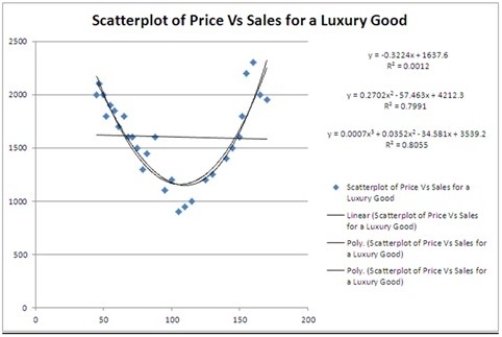
A) 1171.85
B) 1133.10
C) 1106.61
D) 1092.91
Unlock Deck
Unlock for access to all 118 flashcards in this deck.
Unlock Deck
k this deck
53
A model in which the response variable has been log transformed is called a(n) ________.
A) log-log model
B) logarithmic model
C) exponential model
D) linear model
A) log-log model
B) logarithmic model
C) exponential model
D) linear model
Unlock Deck
Unlock for access to all 118 flashcards in this deck.
Unlock Deck
k this deck
54
When the predicted value of the response variable has to be found, in which of the following two models, is there a need for the standard error correction?
A) Linear and log-log
B) Log-log and logarithmic
C) Logarithmic and linear
D) Log-log and exponential
A) Linear and log-log
B) Log-log and logarithmic
C) Logarithmic and linear
D) Log-log and exponential
Unlock Deck
Unlock for access to all 118 flashcards in this deck.
Unlock Deck
k this deck
55
Typically, the sales volume declines with an increase of a product's price. It has been observed, however, that for some luxury goods the sales volume may increase when the price increases. The following scatterplot illustrates this rather unusual relationship.
Using the quadratic equation, predict the sales if the luxury good is priced at $100.
A) 1191.87
B) 1157.64
C) 1160.79
D) 1168.00
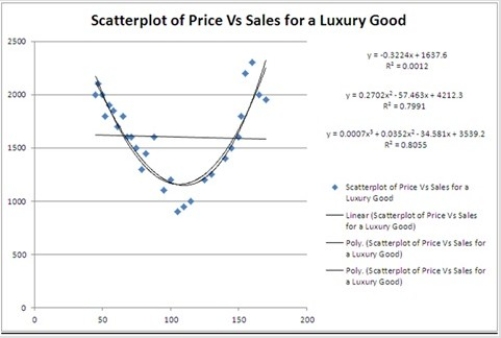
A) 1191.87
B) 1157.64
C) 1160.79
D) 1168.00
Unlock Deck
Unlock for access to all 118 flashcards in this deck.
Unlock Deck
k this deck
56
For which of the following models is
= exp(b0 + b1x +
/2) used to find the predicted value of y ?
A) y = β0 + β1x + ε
B) ln(y) = β0 + β1ln(x ) + ε
C) y = β0 + β1ln(x ) + ε
D) ln(y) = β0 + β1x + ε


A) y = β0 + β1x + ε
B) ln(y) = β0 + β1ln(x ) + ε
C) y = β0 + β1ln(x ) + ε
D) ln(y) = β0 + β1x + ε
Unlock Deck
Unlock for access to all 118 flashcards in this deck.
Unlock Deck
k this deck
57
Typically, the sales volume declines with an increase of a product price. It has been observed, however, that for some luxury goods the sales volume may increase when the price increases. The following scatterplot illustrates this rather unusual relationship.
What is the number of estimated coefficients of the cubic regression model?
A) 1
B) 2
C) 3
D) 4
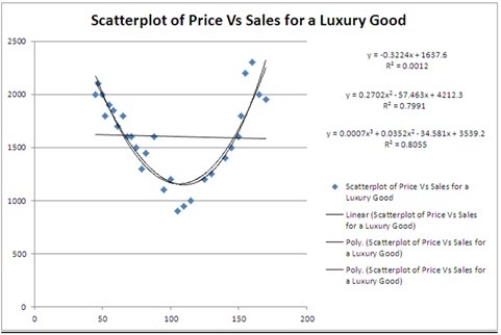
A) 1
B) 2
C) 3
D) 4
Unlock Deck
Unlock for access to all 118 flashcards in this deck.
Unlock Deck
k this deck
58
Typically, the sales volume declines with an increase of a product price. It has been observed, however, that for some luxury goods the sales volume may increase when the price increases. The following scatterplot illustrates this rather unusual relationship.
What can be said about the linear relationship between Price and Sales?
A) The relationship is negatively moderate.
B) There is no relationship.
C) The relationship is positively strong.
D) The relationship is negatively strong.
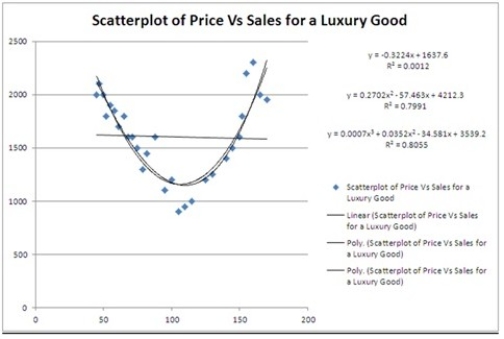
A) The relationship is negatively moderate.
B) There is no relationship.
C) The relationship is positively strong.
D) The relationship is negatively strong.
Unlock Deck
Unlock for access to all 118 flashcards in this deck.
Unlock Deck
k this deck
59
In the model ln(y) = β0 + β1 ln(x) + ε, the coefficient β1 is the approximate ________.
A) change in E(y) when x increases by one unit
B) percentage change in E(y) when x increases by 1%
C) percentage change in E(y) when x increases by one unit
D) change in E(y) when x increases by 1%
A) change in E(y) when x increases by one unit
B) percentage change in E(y) when x increases by 1%
C) percentage change in E(y) when x increases by one unit
D) change in E(y) when x increases by 1%
Unlock Deck
Unlock for access to all 118 flashcards in this deck.
Unlock Deck
k this deck
60
Typically, the sales volume declines with an increase of a product price. It has been observed, however, that for some luxury goods the sales volume may increase when the price increases. The following scatterplot illustrates this rather unusual relationship.
Which of the following models is most likely to be chosen in order to describe the relationship between Price and Sales?
A) Linear
B) Quadratic
C) Cubic
D) Exponential
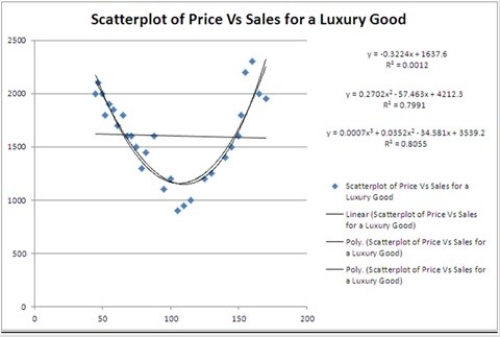
A) Linear
B) Quadratic
C) Cubic
D) Exponential
Unlock Deck
Unlock for access to all 118 flashcards in this deck.
Unlock Deck
k this deck
61
The following data show the cooling temperatures of a freshly brewed cup of coffee after it is poured from the brewing pot into a serving cup. The brewing pot temperature is approximately 180º F.
The output for an exponential model, ln(Temp) = β0 + β1Time + ε, t is below.
How many minutes must elapse after the brewing in order to cool the coffee to 158°F?
A) About five minutes
B) About six minutes
C) About seven minutes
D) About eight minutes

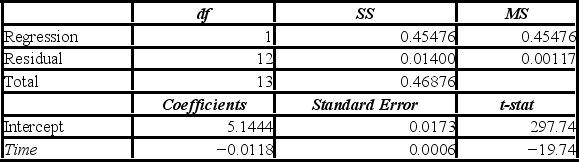
A) About five minutes
B) About six minutes
C) About seven minutes
D) About eight minutes
Unlock Deck
Unlock for access to all 118 flashcards in this deck.
Unlock Deck
k this deck
62
The linear and logarithmic models, y = β0 + β1x + ε and y = β0 + β1 ln(x) + ε, were fit given data on y and x, and the following table summarizes the regression results. Which of the two models provides a better fit? 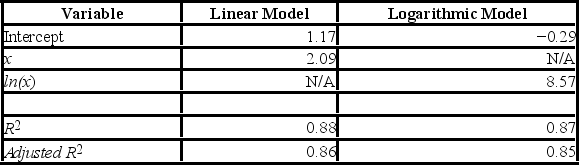
A) The linear model.
B) The logarithmic model.
C) The models are not comparable.
D) The provided information is not sufficient to make the conclusion.
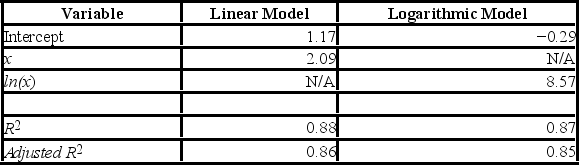
A) The linear model.
B) The logarithmic model.
C) The models are not comparable.
D) The provided information is not sufficient to make the conclusion.
Unlock Deck
Unlock for access to all 118 flashcards in this deck.
Unlock Deck
k this deck
63
The following data, with the corresponding scatterplot, show the average growth rate of Weeping Higan cherry trees planted in Washington, DC. At the time of planting, the trees were one year old and were all six feet in height.
If the age of a tree increases by 1%, then its predicted height increases by approximately ________.
A) 6.1082%
B) 0.06108%
C) 6.1082 feet
D) 0.061082 feet

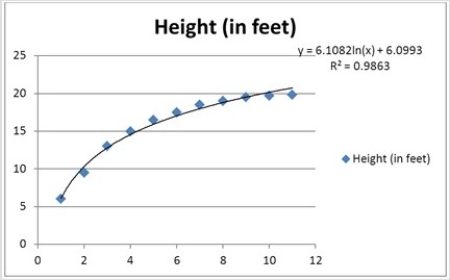
A) 6.1082%
B) 0.06108%
C) 6.1082 feet
D) 0.061082 feet
Unlock Deck
Unlock for access to all 118 flashcards in this deck.
Unlock Deck
k this deck
64
The following data, with the corresponding Excel scatterplot, show the average growth rate of Weeping Higan cherry trees planted in Washington, DC. At the time of planting, the trees were one year old and were all six feet in height.
What percent of the variation in heights is explained by the model? ________.
A) 6.09
B) 6.10
C) 98.63
D) Can't determine from the given information

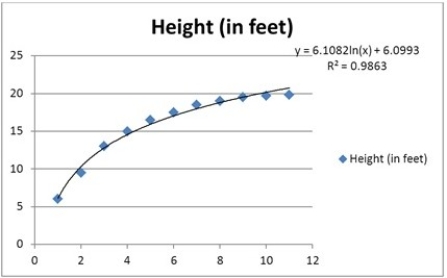
A) 6.09
B) 6.10
C) 98.63
D) Can't determine from the given information
Unlock Deck
Unlock for access to all 118 flashcards in this deck.
Unlock Deck
k this deck
65
The quadratic and logarithmic models, y = β0 + β1x + β2x2 + ε and y = β0 + β1 ln(x) + ε, were fit given data on y and x, and the following table summarizes the regression results. Which of the two models provides a better fit? 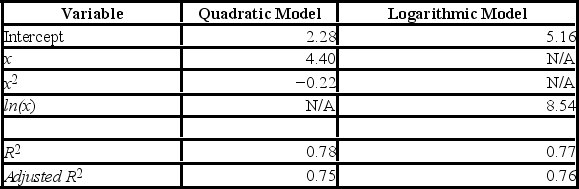
A) The quadratic model.
B) The logarithmic model.
C) The models are not comparable.
D) The provided information is not sufficient to make the conclusion.
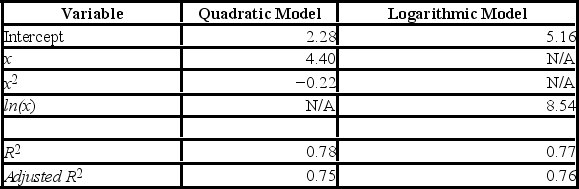
A) The quadratic model.
B) The logarithmic model.
C) The models are not comparable.
D) The provided information is not sufficient to make the conclusion.
Unlock Deck
Unlock for access to all 118 flashcards in this deck.
Unlock Deck
k this deck
66
The following data show the cooling temperatures of a freshly brewed cup of coffee after it is poured from the brewing pot into a serving cup. The brewing pot temperature is approximately 180º F.
The output for an exponential model, ln(Temp) = β0 + β1Time + ε, is below.
Which of the following is the regression equation for making predictions concerning the coffee temperature?
A)
= exp (5.1444 - 0.0118Time
B)
= exp (5.1450 - 0.0118Time
C)
= 5.1444 - 0.0118Time
D)
= 5.1450 - 0.0118Time

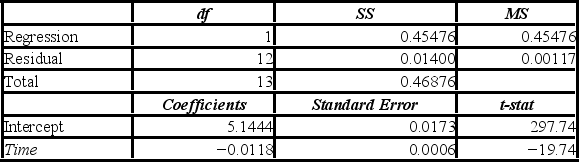
A)

B)

C)

D)

Unlock Deck
Unlock for access to all 118 flashcards in this deck.
Unlock Deck
k this deck
67
The following data show the cooling temperatures of a freshly brewed cup of coffee after it is poured from the brewing pot into a serving cup. The brewing pot temperature is approximately 180º F.
The output for an exponential model, ln(Temp) = β0 + β1Time + ε, is below.
What is the predicted coffee temperature in half an hour after the brewing?
A) 164.72
B) −4.7904
C) 164.74
D) 120.42

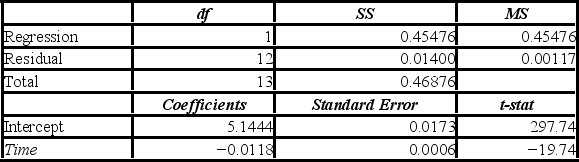
A) 164.72
B) −4.7904
C) 164.74
D) 120.42
Unlock Deck
Unlock for access to all 118 flashcards in this deck.
Unlock Deck
k this deck
68
Which of the following regression models is most likely to provide the best fit for the data represented by the following scatterplot? 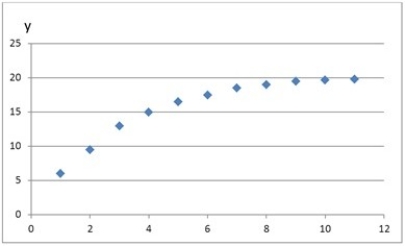
A) Exponential model
B) Logarithmic model
C) Linear model
D) Log-log model
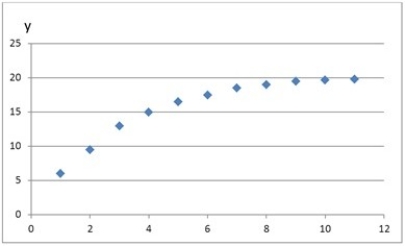
A) Exponential model
B) Logarithmic model
C) Linear model
D) Log-log model
Unlock Deck
Unlock for access to all 118 flashcards in this deck.
Unlock Deck
k this deck
69
Which of the following regression models is most likely to provide the best fit for the data represented by the following scatterplot? 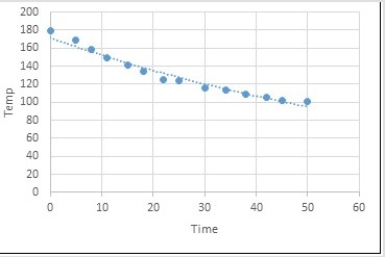
A) Exponential model
B) Logarithmic model
C) Linear model
D) Log-log model
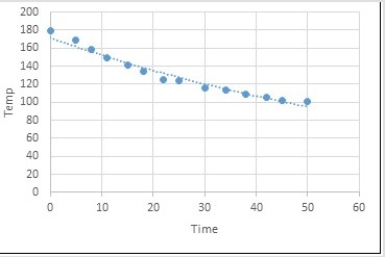
A) Exponential model
B) Logarithmic model
C) Linear model
D) Log-log model
Unlock Deck
Unlock for access to all 118 flashcards in this deck.
Unlock Deck
k this deck
70
The following data, with the corresponding Excel scatterplot, show the average growth rate of Weeping Higan cherry trees planted in Washington, DC. At the time of planting, the trees were one year old and were all six feet in height.
If a cherry tree is planted as a one-year-old and six-foot-tall tree, which of the following is the estimated time needed by the tree to reach 16.5 feet in height?
A) About 4 years
B) About 4.5 years
C) About 5 years
D) About 5.5 years

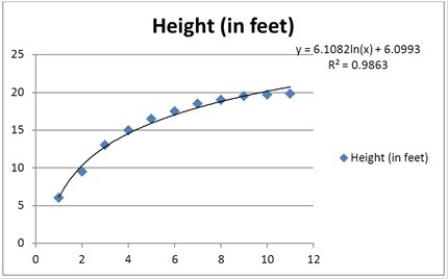
A) About 4 years
B) About 4.5 years
C) About 5 years
D) About 5.5 years
Unlock Deck
Unlock for access to all 118 flashcards in this deck.
Unlock Deck
k this deck
71
The following data show the cooling temperatures of a freshly brewed cup of coffee after it is poured from the brewing pot into a serving cup. The brewing pot temperature is approximately 180º F.
The output for an exponential model, ln(Temp) = β0 + β1Time + ε, is below.
During one minute, the predicted temperature decreases by approximately ________.
A) 0.0118° F
B) 1.18° F
C) 1.18%
D) 11.8%

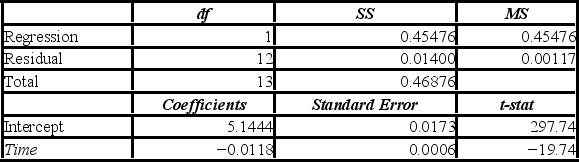
A) 0.0118° F
B) 1.18° F
C) 1.18%
D) 11.8%
Unlock Deck
Unlock for access to all 118 flashcards in this deck.
Unlock Deck
k this deck
72
The log-log and exponential models, ln(x) = β0 + β1ln(x) + ε and ln(y) = β0 + β1x + ε, were fit given data on y and x, and the following table summarizes the regression results. Which of the two models provides a better fit? 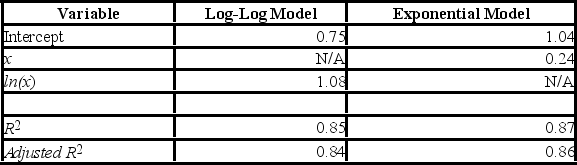
A) The log-log model.
B) The exponential model.
C) The models are not comparable.
D) The provided information is not sufficient to make the conclusion.
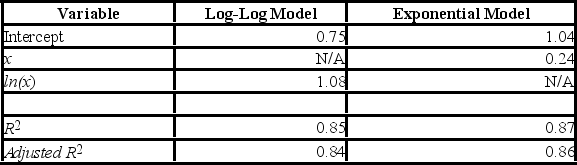
A) The log-log model.
B) The exponential model.
C) The models are not comparable.
D) The provided information is not sufficient to make the conclusion.
Unlock Deck
Unlock for access to all 118 flashcards in this deck.
Unlock Deck
k this deck
73
The logarithmic and log-log models, y = β0 + β1ln(x) + ε and ln(y) = β0 + β1 ln(x) + ε, were fit given data on y and x, and the following table summarizes the regression results. Which of the two models provides a better fit? 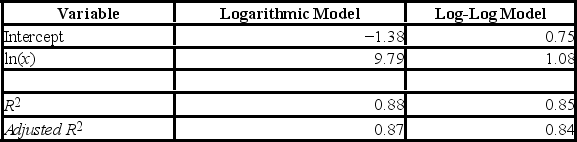
A) The logarithmic model.
B) The log-log model.
C) The models are comparable.
D) The provided information is not sufficient to make the conclusion.
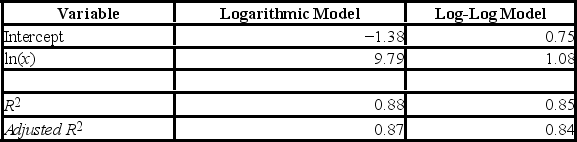
A) The logarithmic model.
B) The log-log model.
C) The models are comparable.
D) The provided information is not sufficient to make the conclusion.
Unlock Deck
Unlock for access to all 118 flashcards in this deck.
Unlock Deck
k this deck
74
The following data, with the corresponding Excel scatterplot, show the average growth rate of Weeping Higan cherry trees planted in Washington, DC. At the time of planting, the trees were one year old and were all six feet in height.
What is the regression model used to describe the relationship between Height and Age?
A) Exponential model
B) Logarithmic model
C) Linear model
D) Log-log model

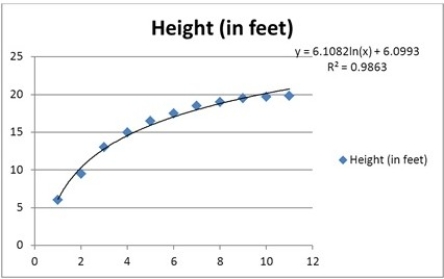
A) Exponential model
B) Logarithmic model
C) Linear model
D) Log-log model
Unlock Deck
Unlock for access to all 118 flashcards in this deck.
Unlock Deck
k this deck
75
The following data, with the corresponding Excel scatterplot, show the average growth rate of Weeping Higan cherry trees planted in Washington, DC. At the time of planting, the trees were one year old and were all six feet in height.
Which of the following is the predicted height of an eight-year-old cherry tree that was planted as a one-year-old and six-foot-tall tree?
A) 54.96
B) 42.66
C) 17.04
D) 18.80

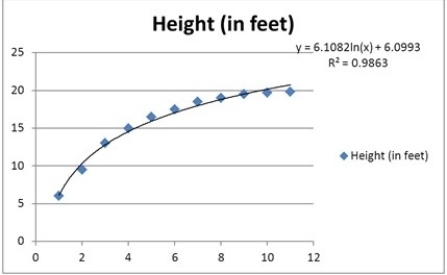
A) 54.96
B) 42.66
C) 17.04
D) 18.80
Unlock Deck
Unlock for access to all 118 flashcards in this deck.
Unlock Deck
k this deck
76
The following data, with the corresponding Excel scatterplot, show the average growth rate of Weeping Higan cherry trees planted in Washington, DC. At the time of planting, the trees were one year old and were all six feet in height.
Which of the following is the correlation coefficient between Height and ln(Age)?
A) −0.9863
B) 0.9863
C) −0.9931
D) 0.9931

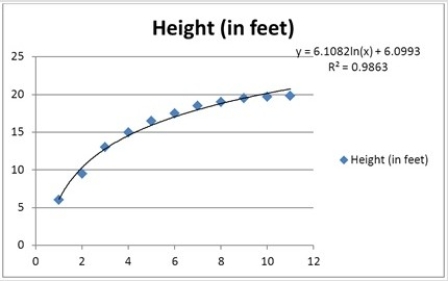
A) −0.9863
B) 0.9863
C) −0.9931
D) 0.9931
Unlock Deck
Unlock for access to all 118 flashcards in this deck.
Unlock Deck
k this deck
77
The following data show the cooling temperatures of a freshly brewed cup of coffee after it is poured from the brewing pot into a serving cup. The brewing pot temperature is approximately 180º F.
The output for an exponential model, ln(Temp) = β0 + β1Time + ε, is below.
Which of the following is the sample correlation coefficient between ln(Temp) and Time?
A) −0.9701
B) 0.9701
C) −0.9849
D) 0.9849

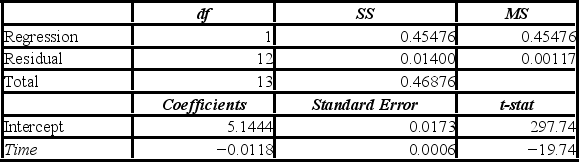
A) −0.9701
B) 0.9701
C) −0.9849
D) 0.9849
Unlock Deck
Unlock for access to all 118 flashcards in this deck.
Unlock Deck
k this deck
78
The following data show the cooling temperatures of a freshly brewed cup of coffee after it is poured from the brewing pot into a serving cup. The brewing pot temperature is approximately 180º F.
The output for an exponential model ln(Temp) = β0 + β1Time + ε, t is below.
Which of the following is the percentage of variation in ln(Temp) explained by the model?
A) 45.48%
B) 97.01%
C) 1.40%
D) 46.88%

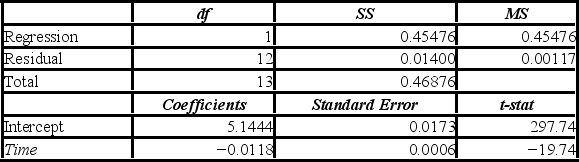
A) 45.48%
B) 97.01%
C) 1.40%
D) 46.88%
Unlock Deck
Unlock for access to all 118 flashcards in this deck.
Unlock Deck
k this deck
79
The following data show the demand for an airline ticket dependent on the price of this ticket.
For the assumed cubic and log-log regression models, Demand = β0 + β1Price + β2Price2 + β3Price3 + ε and ln(Demand) = β0 + β1ln(Price) + ε, the following regression results are available.
Which of the following is the price elasticity of the demand found by the log-log model?
A) 26.3660
B) −3.2577
C) 0.9852
D) 0.2071
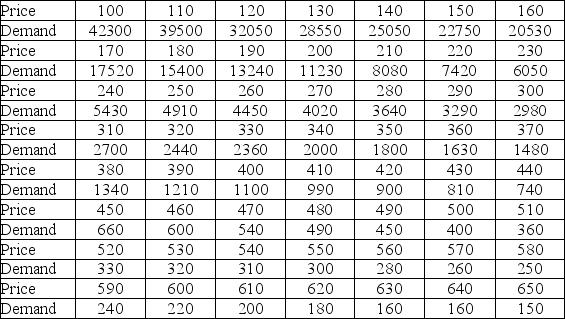
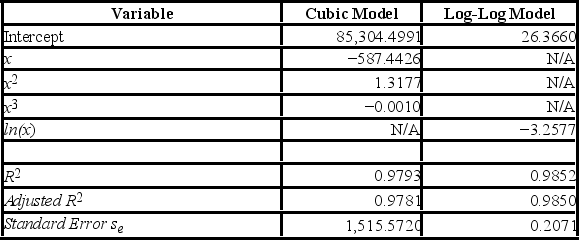
A) 26.3660
B) −3.2577
C) 0.9852
D) 0.2071
Unlock Deck
Unlock for access to all 118 flashcards in this deck.
Unlock Deck
k this deck
80
The following data show the cooling temperatures of a freshly brewed cup of coffee after it is poured from the brewing pot into a serving cup. The brewing pot temperature is approximately 180º F.
The output for an exponential model, ln(Temp) = β0 + β1Time + ε, t is below.
Which of the following is the standard error of the estimate?
A) 0.03421
B) 0.45476
C) 0.00117
D) 0.67436

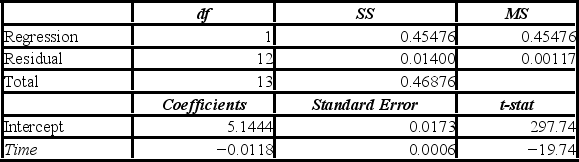
A) 0.03421
B) 0.45476
C) 0.00117
D) 0.67436
Unlock Deck
Unlock for access to all 118 flashcards in this deck.
Unlock Deck
k this deck