Deck 10: Multiple Regression
Question
Question
Question
Question
Question
Question
Question
Question
Question
Question
Question
Question
Question
Question
Question
Question
Question
Question
Question
Question
Question
Question
Question
Question
Question
Question
Question
Question
Question
Question
Question
Question
Question
Question
Question
Question
Question
Question
Question
Question
Question
Question
Question
Question
Question
Question
Question
Question
Question
Question
Question
Question
Question
Question
Question
Question
Question
Question
Question
Question
Question
Question
Question
Question
Question
Question
Question
Question
Question
Question
Question
Question
Unlock Deck
Sign up to unlock the cards in this deck!
Unlock Deck
Unlock Deck
1/72
Play
Full screen (f)
Deck 10: Multiple Regression
1
Use the following
In recent years, fast food restaurants have been required to publish nutrition information about the foods they serve. Nutrition information for a random sample of McDonald's lunch/dinner menu items (excluding sides and drinks) was obtained from their website. Output from a multiple regression analysis is provided.
The regression equation is Calories = 65.2 + 9.46 Total Fat (g) + 0.876 Cholesterol (mg) + 0.131 Sodium (mg)
S = 39.4529 R-Sq = 95.5% R-Sq(adj) = 94.3%
Analysis of Variance

-What are the explanatory variables used in this model?
In recent years, fast food restaurants have been required to publish nutrition information about the foods they serve. Nutrition information for a random sample of McDonald's lunch/dinner menu items (excluding sides and drinks) was obtained from their website. Output from a multiple regression analysis is provided.
The regression equation is Calories = 65.2 + 9.46 Total Fat (g) + 0.876 Cholesterol (mg) + 0.131 Sodium (mg)

S = 39.4529 R-Sq = 95.5% R-Sq(adj) = 94.3%
Analysis of Variance

-What are the explanatory variables used in this model?
Total Fat (g), Cholesterol (mg), and Sodium (mg)
2
Use the following
In recent years, fast food restaurants have been required to publish nutrition information about the foods they serve. Nutrition information for a random sample of McDonald's lunch/dinner menu items (excluding sides and drinks) was obtained from their website. Output from a multiple regression analysis is provided.
The regression equation is Calories = 65.2 + 9.46 Total Fat (g) + 0.876 Cholesterol (mg) + 0.131 Sodium (mg)
S = 39.4529 R-Sq = 95.5% R-Sq(adj) = 94.3%
Analysis of Variance

-Use the provided output to determine how many menu items were included in the sample.
A) 12
B) 13
C) 14
D) 15
In recent years, fast food restaurants have been required to publish nutrition information about the foods they serve. Nutrition information for a random sample of McDonald's lunch/dinner menu items (excluding sides and drinks) was obtained from their website. Output from a multiple regression analysis is provided.
The regression equation is Calories = 65.2 + 9.46 Total Fat (g) + 0.876 Cholesterol (mg) + 0.131 Sodium (mg)

S = 39.4529 R-Sq = 95.5% R-Sq(adj) = 94.3%
Analysis of Variance

-Use the provided output to determine how many menu items were included in the sample.
A) 12
B) 13
C) 14
D) 15
15
3
Use the following
In recent years, fast food restaurants have been required to publish nutrition information about the foods they serve. Nutrition information for a random sample of McDonald's lunch/dinner menu items (excluding sides and drinks) was obtained from their website. Output from a multiple regression analysis is provided.
The regression equation is Calories = 65.2 + 9.46 Total Fat (g) + 0.876 Cholesterol (mg) + 0.131 Sodium (mg)
S = 39.4529 R-Sq = 95.5% R-Sq(adj) = 94.3%
Analysis of Variance

-One of the menu items in the sample is the "McDouble,"
which has 390 calories, 12 grams of fat, 65 mg of cholesterol, and 850 mg of sodium. What is the predicted response for the McDouble? Round your answer to two decimal places.
In recent years, fast food restaurants have been required to publish nutrition information about the foods they serve. Nutrition information for a random sample of McDonald's lunch/dinner menu items (excluding sides and drinks) was obtained from their website. Output from a multiple regression analysis is provided.
The regression equation is Calories = 65.2 + 9.46 Total Fat (g) + 0.876 Cholesterol (mg) + 0.131 Sodium (mg)

S = 39.4529 R-Sq = 95.5% R-Sq(adj) = 94.3%
Analysis of Variance

-One of the menu items in the sample is the "McDouble,"
which has 390 calories, 12 grams of fat, 65 mg of cholesterol, and 850 mg of sodium. What is the predicted response for the McDouble? Round your answer to two decimal places.

4
Use the following
In recent years, fast food restaurants have been required to publish nutrition information about the foods they serve. Nutrition information for a random sample of McDonald's lunch/dinner menu items (excluding sides and drinks) was obtained from their website. Output from a multiple regression analysis is provided.
The regression equation is Calories = 65.2 + 9.46 Total Fat (g) + 0.876 Cholesterol (mg) + 0.131 Sodium (mg)
S = 39.4529 R-Sq = 95.5% R-Sq(adj) = 94.3%
Analysis of Variance

-One of the menu items in the sample is the "McDouble,"
which has 390 calories, 12 grams of fat, 65 mg of cholesterol, and 850 mg of sodium. What is the residual for the McDouble? Round your answer to two decimal places.
In recent years, fast food restaurants have been required to publish nutrition information about the foods they serve. Nutrition information for a random sample of McDonald's lunch/dinner menu items (excluding sides and drinks) was obtained from their website. Output from a multiple regression analysis is provided.
The regression equation is Calories = 65.2 + 9.46 Total Fat (g) + 0.876 Cholesterol (mg) + 0.131 Sodium (mg)

S = 39.4529 R-Sq = 95.5% R-Sq(adj) = 94.3%
Analysis of Variance

-One of the menu items in the sample is the "McDouble,"
which has 390 calories, 12 grams of fat, 65 mg of cholesterol, and 850 mg of sodium. What is the residual for the McDouble? Round your answer to two decimal places.
Unlock Deck
Unlock for access to all 72 flashcards in this deck.
Unlock Deck
k this deck
5
Use the following
In recent years, fast food restaurants have been required to publish nutrition information about the foods they serve. Nutrition information for a random sample of McDonald's lunch/dinner menu items (excluding sides and drinks) was obtained from their website. Output from a multiple regression analysis is provided.
The regression equation is Calories = 65.2 + 9.46 Total Fat (g) + 0.876 Cholesterol (mg) + 0.131 Sodium (mg)
S = 39.4529 R-Sq = 95.5% R-Sq(adj) = 94.3%
Analysis of Variance

-Which predictor appears to be the most important in this model? Explain briefly.
In recent years, fast food restaurants have been required to publish nutrition information about the foods they serve. Nutrition information for a random sample of McDonald's lunch/dinner menu items (excluding sides and drinks) was obtained from their website. Output from a multiple regression analysis is provided.
The regression equation is Calories = 65.2 + 9.46 Total Fat (g) + 0.876 Cholesterol (mg) + 0.131 Sodium (mg)

S = 39.4529 R-Sq = 95.5% R-Sq(adj) = 94.3%
Analysis of Variance

-Which predictor appears to be the most important in this model? Explain briefly.
Unlock Deck
Unlock for access to all 72 flashcards in this deck.
Unlock Deck
k this deck
6
Use the following
In recent years, fast food restaurants have been required to publish nutrition information about the foods they serve. Nutrition information for a random sample of McDonald's lunch/dinner menu items (excluding sides and drinks) was obtained from their website. Output from a multiple regression analysis is provided.
The regression equation is Calories = 65.2 + 9.46 Total Fat (g) + 0.876 Cholesterol (mg) + 0.131 Sodium (mg)
S = 39.4529 R-Sq = 95.5% R-Sq(adj) = 94.3%
Analysis of Variance

-Interpret the coefficient of Sodium in context.
In recent years, fast food restaurants have been required to publish nutrition information about the foods they serve. Nutrition information for a random sample of McDonald's lunch/dinner menu items (excluding sides and drinks) was obtained from their website. Output from a multiple regression analysis is provided.
The regression equation is Calories = 65.2 + 9.46 Total Fat (g) + 0.876 Cholesterol (mg) + 0.131 Sodium (mg)

S = 39.4529 R-Sq = 95.5% R-Sq(adj) = 94.3%
Analysis of Variance

-Interpret the coefficient of Sodium in context.
Unlock Deck
Unlock for access to all 72 flashcards in this deck.
Unlock Deck
k this deck
7
Use the following
In recent years, fast food restaurants have been required to publish nutrition information about the foods they serve. Nutrition information for a random sample of McDonald's lunch/dinner menu items (excluding sides and drinks) was obtained from their website. Output from a multiple regression analysis is provided.
The regression equation is Calories = 65.2 + 9.46 Total Fat (g) + 0.876 Cholesterol (mg) + 0.131 Sodium (mg)
S = 39.4529 R-Sq = 95.5% R-Sq(adj) = 94.3%
Analysis of Variance

-Interpret R2 for this model.
In recent years, fast food restaurants have been required to publish nutrition information about the foods they serve. Nutrition information for a random sample of McDonald's lunch/dinner menu items (excluding sides and drinks) was obtained from their website. Output from a multiple regression analysis is provided.
The regression equation is Calories = 65.2 + 9.46 Total Fat (g) + 0.876 Cholesterol (mg) + 0.131 Sodium (mg)

S = 39.4529 R-Sq = 95.5% R-Sq(adj) = 94.3%
Analysis of Variance

-Interpret R2 for this model.
Unlock Deck
Unlock for access to all 72 flashcards in this deck.
Unlock Deck
k this deck
8
Use the following
In recent years, fast food restaurants have been required to publish nutrition information about the foods they serve. Nutrition information for a random sample of McDonald's lunch/dinner menu items (excluding sides and drinks) was obtained from their website. Output from a multiple regression analysis is provided.
The regression equation is Calories = 65.2 + 9.46 Total Fat (g) + 0.876 Cholesterol (mg) + 0.131 Sodium (mg)
S = 39.4529 R-Sq = 95.5% R-Sq(adj) = 94.3%
Analysis of Variance

-At the 5% significance level, is the model effective according to the ANOVA test? Include all details of the test.
In recent years, fast food restaurants have been required to publish nutrition information about the foods they serve. Nutrition information for a random sample of McDonald's lunch/dinner menu items (excluding sides and drinks) was obtained from their website. Output from a multiple regression analysis is provided.
The regression equation is Calories = 65.2 + 9.46 Total Fat (g) + 0.876 Cholesterol (mg) + 0.131 Sodium (mg)

S = 39.4529 R-Sq = 95.5% R-Sq(adj) = 94.3%
Analysis of Variance

-At the 5% significance level, is the model effective according to the ANOVA test? Include all details of the test.
Unlock Deck
Unlock for access to all 72 flashcards in this deck.
Unlock Deck
k this deck
9
Use the following
In recent years, fast food restaurants have been required to publish nutrition information about the foods they serve. Nutrition information for a random sample of McDonald's lunch/dinner menu items (excluding sides and drinks) was obtained from their website. Output from a multiple regression analysis is provided.
The regression equation is Calories = 65.2 + 9.46 Total Fat (g) + 0.876 Cholesterol (mg) + 0.131 Sodium (mg)
S = 39.4529 R-Sq = 95.5% R-Sq(adj) = 94.3%
Analysis of Variance

-Which predictors are significant at the 5% level? What are their p-values?
In recent years, fast food restaurants have been required to publish nutrition information about the foods they serve. Nutrition information for a random sample of McDonald's lunch/dinner menu items (excluding sides and drinks) was obtained from their website. Output from a multiple regression analysis is provided.
The regression equation is Calories = 65.2 + 9.46 Total Fat (g) + 0.876 Cholesterol (mg) + 0.131 Sodium (mg)

S = 39.4529 R-Sq = 95.5% R-Sq(adj) = 94.3%
Analysis of Variance

-Which predictors are significant at the 5% level? What are their p-values?
Unlock Deck
Unlock for access to all 72 flashcards in this deck.
Unlock Deck
k this deck
10
Use the following
In recent years, fast food restaurants have been required to publish nutrition information about the foods they serve. Nutrition information for a random sample of McDonald's lunch/dinner menu items (excluding sides and drinks) was obtained from their website. Output from a multiple regression analysis is provided.
The regression equation is Calories = 65.2 + 9.46 Total Fat (g) + 0.876 Cholesterol (mg) + 0.131 Sodium (mg)
S = 39.4529 R-Sq = 95.5% R-Sq(adj) = 94.3%
Analysis of Variance

-A boxplot of the residuals and a scatterplot of the residuals versus the predicted values are provided. Discuss whether the conditions for a multiple linear regression are reasonable by referring to the appropriate plots.
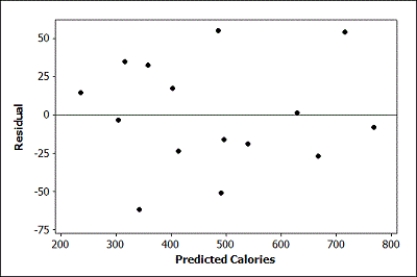
In recent years, fast food restaurants have been required to publish nutrition information about the foods they serve. Nutrition information for a random sample of McDonald's lunch/dinner menu items (excluding sides and drinks) was obtained from their website. Output from a multiple regression analysis is provided.
The regression equation is Calories = 65.2 + 9.46 Total Fat (g) + 0.876 Cholesterol (mg) + 0.131 Sodium (mg)

S = 39.4529 R-Sq = 95.5% R-Sq(adj) = 94.3%
Analysis of Variance

-A boxplot of the residuals and a scatterplot of the residuals versus the predicted values are provided. Discuss whether the conditions for a multiple linear regression are reasonable by referring to the appropriate plots.
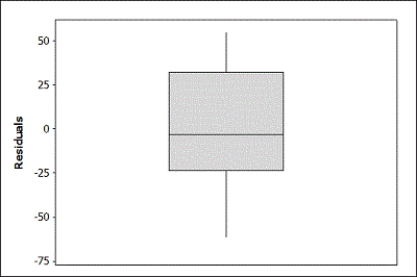
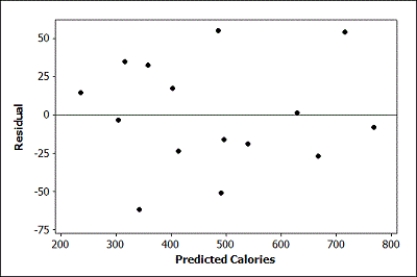
Unlock Deck
Unlock for access to all 72 flashcards in this deck.
Unlock Deck
k this deck
11
Use the following
In recent years, fast food restaurants have been required to publish nutrition information about the foods they serve. Nutrition information for a random sample of McDonald's lunch/dinner menu items (excluding sides and drinks) was obtained from their website. Output from a multiple regression analysis is provided.
The regression equation is Calories = 65.2 + 9.46 Total Fat (g) + 0.876 Cholesterol (mg) + 0.131 Sodium (mg)
S = 39.4529 R-Sq = 95.5% R-Sq(adj) = 94.3%
Analysis of Variance

-Which variable, if any, would you suggest to try eliminating first to possibly improve this model? Describe one way in which you might determine if the model had been improved by removing that variable. Explain briefly.
In recent years, fast food restaurants have been required to publish nutrition information about the foods they serve. Nutrition information for a random sample of McDonald's lunch/dinner menu items (excluding sides and drinks) was obtained from their website. Output from a multiple regression analysis is provided.
The regression equation is Calories = 65.2 + 9.46 Total Fat (g) + 0.876 Cholesterol (mg) + 0.131 Sodium (mg)

S = 39.4529 R-Sq = 95.5% R-Sq(adj) = 94.3%
Analysis of Variance

-Which variable, if any, would you suggest to try eliminating first to possibly improve this model? Describe one way in which you might determine if the model had been improved by removing that variable. Explain briefly.
Unlock Deck
Unlock for access to all 72 flashcards in this deck.
Unlock Deck
k this deck
12
Use the following to answer questions :
Data were collected on the age (in years), mileage (in thousands of miles), and price (in thousands of dollars) of a random sample of used Hyundai Elantras. Output from two models are provided.
Single Predictor Model:
The regression equation is Price = 13.8 - 0.0912 Mileage
Two Predictor Model:
The regression equation is Price = 15.2 - 0.0101 Mileage - 1.55 Age
S = 1.39445 R-Sq = 89.0% R-Sq(adj) = 88.0%
Analysis of Variance

-What is the explanatory variable used in the single predictor model?
Data were collected on the age (in years), mileage (in thousands of miles), and price (in thousands of dollars) of a random sample of used Hyundai Elantras. Output from two models are provided.
Single Predictor Model:
The regression equation is Price = 13.8 - 0.0912 Mileage

Two Predictor Model:
The regression equation is Price = 15.2 - 0.0101 Mileage - 1.55 Age

S = 1.39445 R-Sq = 89.0% R-Sq(adj) = 88.0%
Analysis of Variance

-What is the explanatory variable used in the single predictor model?
Unlock Deck
Unlock for access to all 72 flashcards in this deck.
Unlock Deck
k this deck
13
Use the following to answer questions :
Data were collected on the age (in years), mileage (in thousands of miles), and price (in thousands of dollars) of a random sample of used Hyundai Elantras. Output from two models are provided.
Single Predictor Model:
The regression equation is Price = 13.8 - 0.0912 Mileage
Two Predictor Model:
The regression equation is Price = 15.2 - 0.0101 Mileage - 1.55 Age
S = 1.39445 R-Sq = 89.0% R-Sq(adj) = 88.0%
Analysis of Variance

-One of the cars in the sample was a 5-year-old Hyundai Elantra with 87,100 miles being sold for $6,000. What is the predicted price of this car using the single predictor model? Round to three decimal places.
Data were collected on the age (in years), mileage (in thousands of miles), and price (in thousands of dollars) of a random sample of used Hyundai Elantras. Output from two models are provided.
Single Predictor Model:
The regression equation is Price = 13.8 - 0.0912 Mileage

Two Predictor Model:
The regression equation is Price = 15.2 - 0.0101 Mileage - 1.55 Age

S = 1.39445 R-Sq = 89.0% R-Sq(adj) = 88.0%
Analysis of Variance

-One of the cars in the sample was a 5-year-old Hyundai Elantra with 87,100 miles being sold for $6,000. What is the predicted price of this car using the single predictor model? Round to three decimal places.
Unlock Deck
Unlock for access to all 72 flashcards in this deck.
Unlock Deck
k this deck
14
Use the following to answer questions :
Data were collected on the age (in years), mileage (in thousands of miles), and price (in thousands of dollars) of a random sample of used Hyundai Elantras. Output from two models are provided.
Single Predictor Model:
The regression equation is Price = 13.8 - 0.0912 Mileage
Two Predictor Model:
The regression equation is Price = 15.2 - 0.0101 Mileage - 1.55 Age
S = 1.39445 R-Sq = 89.0% R-Sq(adj) = 88.0%
Analysis of Variance

-One of the cars in the sample was a 5-year-old Hyundai Elantra with 87,100 miles being sold for $6,000. What is the predicted price of the car using the two predictor model? Round to three decimal places.
Data were collected on the age (in years), mileage (in thousands of miles), and price (in thousands of dollars) of a random sample of used Hyundai Elantras. Output from two models are provided.
Single Predictor Model:
The regression equation is Price = 13.8 - 0.0912 Mileage

Two Predictor Model:
The regression equation is Price = 15.2 - 0.0101 Mileage - 1.55 Age

S = 1.39445 R-Sq = 89.0% R-Sq(adj) = 88.0%
Analysis of Variance

-One of the cars in the sample was a 5-year-old Hyundai Elantra with 87,100 miles being sold for $6,000. What is the predicted price of the car using the two predictor model? Round to three decimal places.
Unlock Deck
Unlock for access to all 72 flashcards in this deck.
Unlock Deck
k this deck
15
Use the following to answer questions :
Data were collected on the age (in years), mileage (in thousands of miles), and price (in thousands of dollars) of a random sample of used Hyundai Elantras. Output from two models are provided.
Single Predictor Model:
The regression equation is Price = 13.8 - 0.0912 Mileage
Two Predictor Model:
The regression equation is Price = 15.2 - 0.0101 Mileage - 1.55 Age
S = 1.39445 R-Sq = 89.0% R-Sq(adj) = 88.0%
Analysis of Variance

-Is mileage a significant single predictor of the price of used Hyundai Elantras? Use
= 0.05. Include all details of your test.
Data were collected on the age (in years), mileage (in thousands of miles), and price (in thousands of dollars) of a random sample of used Hyundai Elantras. Output from two models are provided.
Single Predictor Model:
The regression equation is Price = 13.8 - 0.0912 Mileage

Two Predictor Model:
The regression equation is Price = 15.2 - 0.0101 Mileage - 1.55 Age

S = 1.39445 R-Sq = 89.0% R-Sq(adj) = 88.0%
Analysis of Variance

-Is mileage a significant single predictor of the price of used Hyundai Elantras? Use

Unlock Deck
Unlock for access to all 72 flashcards in this deck.
Unlock Deck
k this deck
16
Use the following to answer questions :
Data were collected on the age (in years), mileage (in thousands of miles), and price (in thousands of dollars) of a random sample of used Hyundai Elantras. Output from two models are provided.
Single Predictor Model:
The regression equation is Price = 13.8 - 0.0912 Mileage
Two Predictor Model:
The regression equation is Price = 15.2 - 0.0101 Mileage - 1.55 Age
S = 1.39445 R-Sq = 89.0% R-Sq(adj) = 88.0%
Analysis of Variance

-Explain why Age is a potential confounding variable in the relationship between Age and Price of used Hyundai Elantras.
Data were collected on the age (in years), mileage (in thousands of miles), and price (in thousands of dollars) of a random sample of used Hyundai Elantras. Output from two models are provided.
Single Predictor Model:
The regression equation is Price = 13.8 - 0.0912 Mileage

Two Predictor Model:
The regression equation is Price = 15.2 - 0.0101 Mileage - 1.55 Age

S = 1.39445 R-Sq = 89.0% R-Sq(adj) = 88.0%
Analysis of Variance

-Explain why Age is a potential confounding variable in the relationship between Age and Price of used Hyundai Elantras.
Unlock Deck
Unlock for access to all 72 flashcards in this deck.
Unlock Deck
k this deck
17
Use the following to answer questions :
Data were collected on the age (in years), mileage (in thousands of miles), and price (in thousands of dollars) of a random sample of used Hyundai Elantras. Output from two models are provided.
Single Predictor Model:
The regression equation is Price = 13.8 - 0.0912 Mileage
Two Predictor Model:
The regression equation is Price = 15.2 - 0.0101 Mileage - 1.55 Age
S = 1.39445 R-Sq = 89.0% R-Sq(adj) = 88.0%
Analysis of Variance

-Is the two predictor model effective according to the ANOVA test? Use
= 0.05. Include all details of the test.
Data were collected on the age (in years), mileage (in thousands of miles), and price (in thousands of dollars) of a random sample of used Hyundai Elantras. Output from two models are provided.
Single Predictor Model:
The regression equation is Price = 13.8 - 0.0912 Mileage

Two Predictor Model:
The regression equation is Price = 15.2 - 0.0101 Mileage - 1.55 Age

S = 1.39445 R-Sq = 89.0% R-Sq(adj) = 88.0%
Analysis of Variance

-Is the two predictor model effective according to the ANOVA test? Use

Unlock Deck
Unlock for access to all 72 flashcards in this deck.
Unlock Deck
k this deck
18
Use the following to answer questions :
Data were collected on the age (in years), mileage (in thousands of miles), and price (in thousands of dollars) of a random sample of used Hyundai Elantras. Output from two models are provided.
Single Predictor Model:
The regression equation is Price = 13.8 - 0.0912 Mileage
Two Predictor Model:
The regression equation is Price = 15.2 - 0.0101 Mileage - 1.55 Age
S = 1.39445 R-Sq = 89.0% R-Sq(adj) = 88.0%
Analysis of Variance

-Is mileage a significant predictor of the price of used Hyundai Elantras, even after accounting for age? Explain briefly.
Data were collected on the age (in years), mileage (in thousands of miles), and price (in thousands of dollars) of a random sample of used Hyundai Elantras. Output from two models are provided.
Single Predictor Model:
The regression equation is Price = 13.8 - 0.0912 Mileage

Two Predictor Model:
The regression equation is Price = 15.2 - 0.0101 Mileage - 1.55 Age

S = 1.39445 R-Sq = 89.0% R-Sq(adj) = 88.0%
Analysis of Variance

-Is mileage a significant predictor of the price of used Hyundai Elantras, even after accounting for age? Explain briefly.
Unlock Deck
Unlock for access to all 72 flashcards in this deck.
Unlock Deck
k this deck
19
Use the following to answer questions :
Data were collected on the age (in years), mileage (in thousands of miles), and price (in thousands of dollars) of a random sample of used Hyundai Elantras. Output from two models are provided.
Single Predictor Model:
The regression equation is Price = 13.8 - 0.0912 Mileage
Two Predictor Model:
The regression equation is Price = 15.2 - 0.0101 Mileage - 1.55 Age
S = 1.39445 R-Sq = 89.0% R-Sq(adj) = 88.0%
Analysis of Variance

-Use the provided output to determine how many cars were in the sample.
A) 22
B) 23
C) 24
D) 25
Data were collected on the age (in years), mileage (in thousands of miles), and price (in thousands of dollars) of a random sample of used Hyundai Elantras. Output from two models are provided.
Single Predictor Model:
The regression equation is Price = 13.8 - 0.0912 Mileage

Two Predictor Model:
The regression equation is Price = 15.2 - 0.0101 Mileage - 1.55 Age

S = 1.39445 R-Sq = 89.0% R-Sq(adj) = 88.0%
Analysis of Variance

-Use the provided output to determine how many cars were in the sample.
A) 22
B) 23
C) 24
D) 25
Unlock Deck
Unlock for access to all 72 flashcards in this deck.
Unlock Deck
k this deck
20
Use the following to answer questions :
Data were collected on the age (in years), mileage (in thousands of miles), and price (in thousands of dollars) of a random sample of used Hyundai Elantras. Output from two models are provided.
Single Predictor Model:
The regression equation is Price = 13.8 - 0.0912 Mileage
Two Predictor Model:
The regression equation is Price = 15.2 - 0.0101 Mileage - 1.55 Age
S = 1.39445 R-Sq = 89.0% R-Sq(adj) = 88.0%
Analysis of Variance

-A boxplot of the residuals and a scatterplot of the residuals versus the predicted values from the two predictor model are provided. Discuss whether the conditions for a multiple linear regression are reasonable by referring to the appropriate plots.
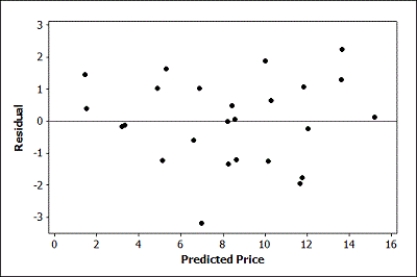
Data were collected on the age (in years), mileage (in thousands of miles), and price (in thousands of dollars) of a random sample of used Hyundai Elantras. Output from two models are provided.
Single Predictor Model:
The regression equation is Price = 13.8 - 0.0912 Mileage

Two Predictor Model:
The regression equation is Price = 15.2 - 0.0101 Mileage - 1.55 Age

S = 1.39445 R-Sq = 89.0% R-Sq(adj) = 88.0%
Analysis of Variance

-A boxplot of the residuals and a scatterplot of the residuals versus the predicted values from the two predictor model are provided. Discuss whether the conditions for a multiple linear regression are reasonable by referring to the appropriate plots.
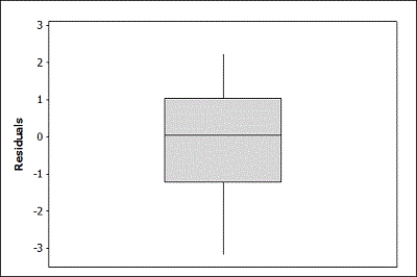
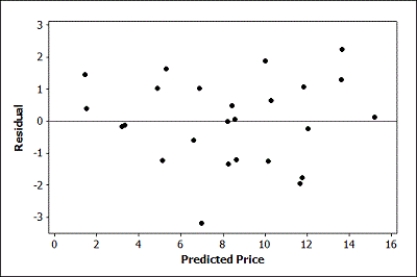
Unlock Deck
Unlock for access to all 72 flashcards in this deck.
Unlock Deck
k this deck
21
Use the following to answer questions :
Data were collected on the age (in years), mileage (in thousands of miles), and price (in thousands of dollars) of a random sample of used Hyundai Elantras. Output from two models are provided.
Single Predictor Model:
The regression equation is Price = 13.8 - 0.0912 Mileage
Two Predictor Model:
The regression equation is Price = 15.2 - 0.0101 Mileage - 1.55 Age
S = 1.39445 R-Sq = 89.0% R-Sq(adj) = 88.0%
Analysis of Variance

-Regression output for the model that only uses Age as a predictor in the model is provided. Assuming that the residuals for this single predictor model do not indicate any problems, is this model an improvement over the model that uses both Age and Mileage as predictors? Statistically justify your answer by discussing at least two quantitative criteria.
The regression equation is Price = 15.3 - 1.71 Age
S = 1.37179 R-Sq = 88.9% R-Sq(adj) = 88.4%
Analysis of Variance

Data were collected on the age (in years), mileage (in thousands of miles), and price (in thousands of dollars) of a random sample of used Hyundai Elantras. Output from two models are provided.
Single Predictor Model:
The regression equation is Price = 13.8 - 0.0912 Mileage

Two Predictor Model:
The regression equation is Price = 15.2 - 0.0101 Mileage - 1.55 Age

S = 1.39445 R-Sq = 89.0% R-Sq(adj) = 88.0%
Analysis of Variance

-Regression output for the model that only uses Age as a predictor in the model is provided. Assuming that the residuals for this single predictor model do not indicate any problems, is this model an improvement over the model that uses both Age and Mileage as predictors? Statistically justify your answer by discussing at least two quantitative criteria.
The regression equation is Price = 15.3 - 1.71 Age

S = 1.37179 R-Sq = 88.9% R-Sq(adj) = 88.4%
Analysis of Variance

Unlock Deck
Unlock for access to all 72 flashcards in this deck.
Unlock Deck
k this deck
22
Use the following to answer questions :
A quantitatively savvy, young couple is interested in purchasing a home in northern New York. They collected data on houses that had recently sold in the two towns they are considering. The variables they collected are the selling price of the home (in thousands of dollars), the size of the home (in square feet), the age of the home (in years), and the town in which the house is located (coded 1 = Canton and 0 = Potsdam). Output from their multiple regression analysis is provided.
The regression equation is
Price (in thousands) = 69.2 + 0.0627 Size (sq. ft.) - 0.632 Age + 1.6 Town
S = 40.0763 R-Sq = 59.3% R-Sq(adj) = 56.5%
Analysis of Variance

-One of the houses they are considering is a 92 year old, 1,742 square foot house in Canton. What is the predicted selling price of this house? Round to three decimal places.
A quantitatively savvy, young couple is interested in purchasing a home in northern New York. They collected data on houses that had recently sold in the two towns they are considering. The variables they collected are the selling price of the home (in thousands of dollars), the size of the home (in square feet), the age of the home (in years), and the town in which the house is located (coded 1 = Canton and 0 = Potsdam). Output from their multiple regression analysis is provided.
The regression equation is
Price (in thousands) = 69.2 + 0.0627 Size (sq. ft.) - 0.632 Age + 1.6 Town

S = 40.0763 R-Sq = 59.3% R-Sq(adj) = 56.5%
Analysis of Variance

-One of the houses they are considering is a 92 year old, 1,742 square foot house in Canton. What is the predicted selling price of this house? Round to three decimal places.
Unlock Deck
Unlock for access to all 72 flashcards in this deck.
Unlock Deck
k this deck
23
Use the following to answer questions :
A quantitatively savvy, young couple is interested in purchasing a home in northern New York. They collected data on houses that had recently sold in the two towns they are considering. The variables they collected are the selling price of the home (in thousands of dollars), the size of the home (in square feet), the age of the home (in years), and the town in which the house is located (coded 1 = Canton and 0 = Potsdam). Output from their multiple regression analysis is provided.
The regression equation is
Price (in thousands) = 69.2 + 0.0627 Size (sq. ft.) - 0.632 Age + 1.6 Town
S = 40.0763 R-Sq = 59.3% R-Sq(adj) = 56.5%
Analysis of Variance

-One of the houses they are considering is a 62 year old, 1,865 square foot house in Potsdam. What is the predicted selling price of this house? Round to three decimal places.
A quantitatively savvy, young couple is interested in purchasing a home in northern New York. They collected data on houses that had recently sold in the two towns they are considering. The variables they collected are the selling price of the home (in thousands of dollars), the size of the home (in square feet), the age of the home (in years), and the town in which the house is located (coded 1 = Canton and 0 = Potsdam). Output from their multiple regression analysis is provided.
The regression equation is
Price (in thousands) = 69.2 + 0.0627 Size (sq. ft.) - 0.632 Age + 1.6 Town

S = 40.0763 R-Sq = 59.3% R-Sq(adj) = 56.5%
Analysis of Variance

-One of the houses they are considering is a 62 year old, 1,865 square foot house in Potsdam. What is the predicted selling price of this house? Round to three decimal places.
Unlock Deck
Unlock for access to all 72 flashcards in this deck.
Unlock Deck
k this deck
24
Use the following to answer questions :
A quantitatively savvy, young couple is interested in purchasing a home in northern New York. They collected data on houses that had recently sold in the two towns they are considering. The variables they collected are the selling price of the home (in thousands of dollars), the size of the home (in square feet), the age of the home (in years), and the town in which the house is located (coded 1 = Canton and 0 = Potsdam). Output from their multiple regression analysis is provided.
The regression equation is
Price (in thousands) = 69.2 + 0.0627 Size (sq. ft.) - 0.632 Age + 1.6 Town
S = 40.0763 R-Sq = 59.3% R-Sq(adj) = 56.5%
Analysis of Variance

-Interpret the coefficient of Age in context.
A quantitatively savvy, young couple is interested in purchasing a home in northern New York. They collected data on houses that had recently sold in the two towns they are considering. The variables they collected are the selling price of the home (in thousands of dollars), the size of the home (in square feet), the age of the home (in years), and the town in which the house is located (coded 1 = Canton and 0 = Potsdam). Output from their multiple regression analysis is provided.
The regression equation is
Price (in thousands) = 69.2 + 0.0627 Size (sq. ft.) - 0.632 Age + 1.6 Town

S = 40.0763 R-Sq = 59.3% R-Sq(adj) = 56.5%
Analysis of Variance

-Interpret the coefficient of Age in context.
Unlock Deck
Unlock for access to all 72 flashcards in this deck.
Unlock Deck
k this deck
25
Use the following to answer questions :
A quantitatively savvy, young couple is interested in purchasing a home in northern New York. They collected data on houses that had recently sold in the two towns they are considering. The variables they collected are the selling price of the home (in thousands of dollars), the size of the home (in square feet), the age of the home (in years), and the town in which the house is located (coded 1 = Canton and 0 = Potsdam). Output from their multiple regression analysis is provided.
The regression equation is
Price (in thousands) = 69.2 + 0.0627 Size (sq. ft.) - 0.632 Age + 1.6 Town
S = 40.0763 R-Sq = 59.3% R-Sq(adj) = 56.5%
Analysis of Variance

-Interpret the coefficient of Town in context.
A quantitatively savvy, young couple is interested in purchasing a home in northern New York. They collected data on houses that had recently sold in the two towns they are considering. The variables they collected are the selling price of the home (in thousands of dollars), the size of the home (in square feet), the age of the home (in years), and the town in which the house is located (coded 1 = Canton and 0 = Potsdam). Output from their multiple regression analysis is provided.
The regression equation is
Price (in thousands) = 69.2 + 0.0627 Size (sq. ft.) - 0.632 Age + 1.6 Town

S = 40.0763 R-Sq = 59.3% R-Sq(adj) = 56.5%
Analysis of Variance

-Interpret the coefficient of Town in context.
Unlock Deck
Unlock for access to all 72 flashcards in this deck.
Unlock Deck
k this deck
26
Use the following to answer questions :
A quantitatively savvy, young couple is interested in purchasing a home in northern New York. They collected data on houses that had recently sold in the two towns they are considering. The variables they collected are the selling price of the home (in thousands of dollars), the size of the home (in square feet), the age of the home (in years), and the town in which the house is located (coded 1 = Canton and 0 = Potsdam). Output from their multiple regression analysis is provided.
The regression equation is
Price (in thousands) = 69.2 + 0.0627 Size (sq. ft.) - 0.632 Age + 1.6 Town
S = 40.0763 R-Sq = 59.3% R-Sq(adj) = 56.5%
Analysis of Variance

-How many houses are used in this dataset?
A) 48
B) 47
C) 46
D) 45
A quantitatively savvy, young couple is interested in purchasing a home in northern New York. They collected data on houses that had recently sold in the two towns they are considering. The variables they collected are the selling price of the home (in thousands of dollars), the size of the home (in square feet), the age of the home (in years), and the town in which the house is located (coded 1 = Canton and 0 = Potsdam). Output from their multiple regression analysis is provided.
The regression equation is
Price (in thousands) = 69.2 + 0.0627 Size (sq. ft.) - 0.632 Age + 1.6 Town

S = 40.0763 R-Sq = 59.3% R-Sq(adj) = 56.5%
Analysis of Variance

-How many houses are used in this dataset?
A) 48
B) 47
C) 46
D) 45
Unlock Deck
Unlock for access to all 72 flashcards in this deck.
Unlock Deck
k this deck
27
Use the following to answer questions :
A quantitatively savvy, young couple is interested in purchasing a home in northern New York. They collected data on houses that had recently sold in the two towns they are considering. The variables they collected are the selling price of the home (in thousands of dollars), the size of the home (in square feet), the age of the home (in years), and the town in which the house is located (coded 1 = Canton and 0 = Potsdam). Output from their multiple regression analysis is provided.
The regression equation is
Price (in thousands) = 69.2 + 0.0627 Size (sq. ft.) - 0.632 Age + 1.6 Town
S = 40.0763 R-Sq = 59.3% R-Sq(adj) = 56.5%
Analysis of Variance

-Interpret R2 for this model.
A quantitatively savvy, young couple is interested in purchasing a home in northern New York. They collected data on houses that had recently sold in the two towns they are considering. The variables they collected are the selling price of the home (in thousands of dollars), the size of the home (in square feet), the age of the home (in years), and the town in which the house is located (coded 1 = Canton and 0 = Potsdam). Output from their multiple regression analysis is provided.
The regression equation is
Price (in thousands) = 69.2 + 0.0627 Size (sq. ft.) - 0.632 Age + 1.6 Town

S = 40.0763 R-Sq = 59.3% R-Sq(adj) = 56.5%
Analysis of Variance

-Interpret R2 for this model.
Unlock Deck
Unlock for access to all 72 flashcards in this deck.
Unlock Deck
k this deck
28
Use the following to answer questions :
A quantitatively savvy, young couple is interested in purchasing a home in northern New York. They collected data on houses that had recently sold in the two towns they are considering. The variables they collected are the selling price of the home (in thousands of dollars), the size of the home (in square feet), the age of the home (in years), and the town in which the house is located (coded 1 = Canton and 0 = Potsdam). Output from their multiple regression analysis is provided.
The regression equation is
Price (in thousands) = 69.2 + 0.0627 Size (sq. ft.) - 0.632 Age + 1.6 Town
S = 40.0763 R-Sq = 59.3% R-Sq(adj) = 56.5%
Analysis of Variance

-Using
= 0.05, is the model effective according to the ANOVA test? Include all details of the test.
A quantitatively savvy, young couple is interested in purchasing a home in northern New York. They collected data on houses that had recently sold in the two towns they are considering. The variables they collected are the selling price of the home (in thousands of dollars), the size of the home (in square feet), the age of the home (in years), and the town in which the house is located (coded 1 = Canton and 0 = Potsdam). Output from their multiple regression analysis is provided.
The regression equation is
Price (in thousands) = 69.2 + 0.0627 Size (sq. ft.) - 0.632 Age + 1.6 Town

S = 40.0763 R-Sq = 59.3% R-Sq(adj) = 56.5%
Analysis of Variance

-Using

Unlock Deck
Unlock for access to all 72 flashcards in this deck.
Unlock Deck
k this deck
29
Use the following to answer questions :
A quantitatively savvy, young couple is interested in purchasing a home in northern New York. They collected data on houses that had recently sold in the two towns they are considering. The variables they collected are the selling price of the home (in thousands of dollars), the size of the home (in square feet), the age of the home (in years), and the town in which the house is located (coded 1 = Canton and 0 = Potsdam). Output from their multiple regression analysis is provided.
The regression equation is
Price (in thousands) = 69.2 + 0.0627 Size (sq. ft.) - 0.632 Age + 1.6 Town
S = 40.0763 R-Sq = 59.3% R-Sq(adj) = 56.5%
Analysis of Variance

-Which predictors are significant at the 5% level? What are their p-values?
A quantitatively savvy, young couple is interested in purchasing a home in northern New York. They collected data on houses that had recently sold in the two towns they are considering. The variables they collected are the selling price of the home (in thousands of dollars), the size of the home (in square feet), the age of the home (in years), and the town in which the house is located (coded 1 = Canton and 0 = Potsdam). Output from their multiple regression analysis is provided.
The regression equation is
Price (in thousands) = 69.2 + 0.0627 Size (sq. ft.) - 0.632 Age + 1.6 Town

S = 40.0763 R-Sq = 59.3% R-Sq(adj) = 56.5%
Analysis of Variance

-Which predictors are significant at the 5% level? What are their p-values?
Unlock Deck
Unlock for access to all 72 flashcards in this deck.
Unlock Deck
k this deck
30
Use the following to answer questions :
A quantitatively savvy, young couple is interested in purchasing a home in northern New York. They collected data on houses that had recently sold in the two towns they are considering. The variables they collected are the selling price of the home (in thousands of dollars), the size of the home (in square feet), the age of the home (in years), and the town in which the house is located (coded 1 = Canton and 0 = Potsdam). Output from their multiple regression analysis is provided.
The regression equation is
Price (in thousands) = 69.2 + 0.0627 Size (sq. ft.) - 0.632 Age + 1.6 Town
S = 40.0763 R-Sq = 59.3% R-Sq(adj) = 56.5%
Analysis of Variance

-A dotplot of the residuals and a scatterplot of the residuals versus the predicted values are provided. Discuss whether the conditions for a multiple linear regression are reasonable by referring to the appropriate plots.
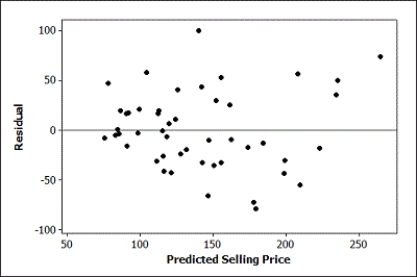
A quantitatively savvy, young couple is interested in purchasing a home in northern New York. They collected data on houses that had recently sold in the two towns they are considering. The variables they collected are the selling price of the home (in thousands of dollars), the size of the home (in square feet), the age of the home (in years), and the town in which the house is located (coded 1 = Canton and 0 = Potsdam). Output from their multiple regression analysis is provided.
The regression equation is
Price (in thousands) = 69.2 + 0.0627 Size (sq. ft.) - 0.632 Age + 1.6 Town

S = 40.0763 R-Sq = 59.3% R-Sq(adj) = 56.5%
Analysis of Variance

-A dotplot of the residuals and a scatterplot of the residuals versus the predicted values are provided. Discuss whether the conditions for a multiple linear regression are reasonable by referring to the appropriate plots.
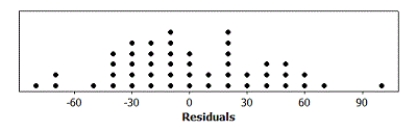
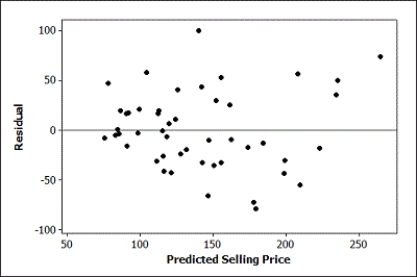
Unlock Deck
Unlock for access to all 72 flashcards in this deck.
Unlock Deck
k this deck
31
Use the following to answer questions :
A quantitatively savvy, young couple is interested in purchasing a home in northern New York. They collected data on houses that had recently sold in the two towns they are considering. The variables they collected are the selling price of the home (in thousands of dollars), the size of the home (in square feet), the age of the home (in years), and the town in which the house is located (coded 1 = Canton and 0 = Potsdam). Output from their multiple regression analysis is provided.
The regression equation is
Price (in thousands) = 69.2 + 0.0627 Size (sq. ft.) - 0.632 Age + 1.6 Town
S = 40.0763 R-Sq = 59.3% R-Sq(adj) = 56.5%
Analysis of Variance

-Regression output for a model that omits Town as a predictor is provided. Assuming that the residuals for this reduced model do not indicate any problems with using multiple regression, is this model an improvement over the model that uses Size, Age, and Town as predictors? Statistically justify your answer by discussing at least two quantitative criteria.
The regression equation is
Price (in thousands) = 70.6 + 0.0624 Size (sq. ft.) - 0.635 Age
S = 39.6368 R-Sq = 59.3% R-Sq(adj) = 57.5%
Analysis of Variance

A quantitatively savvy, young couple is interested in purchasing a home in northern New York. They collected data on houses that had recently sold in the two towns they are considering. The variables they collected are the selling price of the home (in thousands of dollars), the size of the home (in square feet), the age of the home (in years), and the town in which the house is located (coded 1 = Canton and 0 = Potsdam). Output from their multiple regression analysis is provided.
The regression equation is
Price (in thousands) = 69.2 + 0.0627 Size (sq. ft.) - 0.632 Age + 1.6 Town

S = 40.0763 R-Sq = 59.3% R-Sq(adj) = 56.5%
Analysis of Variance

-Regression output for a model that omits Town as a predictor is provided. Assuming that the residuals for this reduced model do not indicate any problems with using multiple regression, is this model an improvement over the model that uses Size, Age, and Town as predictors? Statistically justify your answer by discussing at least two quantitative criteria.
The regression equation is
Price (in thousands) = 70.6 + 0.0624 Size (sq. ft.) - 0.635 Age

S = 39.6368 R-Sq = 59.3% R-Sq(adj) = 57.5%
Analysis of Variance

Unlock Deck
Unlock for access to all 72 flashcards in this deck.
Unlock Deck
k this deck
32
Use the following
While many people count calories, some often don't think about calories in the beverages they consume. Starbucks, one of the leading coffeehouse chains, provides nutrition information about all of their beverages on their website. Nutrition information, including number of calories, fat (g), carbohydrates (g), and protein (g), was collected on a random sample of Starbucks' 16 ounce ("Grande") hot espresso drinks. Note that all of the drinks in the sample are made with 2% milk unless the name specifically included the term "Skinny,"
which is how Starbucks indicated a beverage made with nonfat milk.
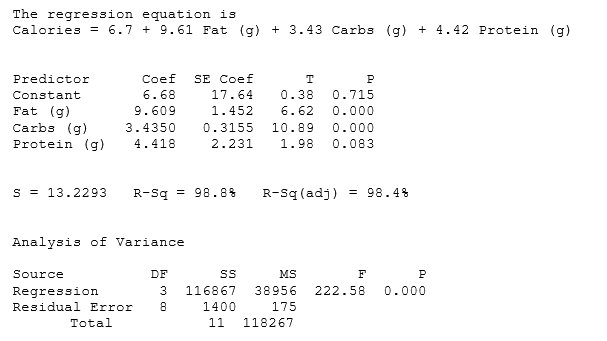
-The "Caramel Macchiato"
was one of the drinks selected for the sample. When made with 2% milk, a grande Caramel Macchiato has 7 grams of fat, 34 grams of carbohydrates, and 10 grams of protein. Predict the number of calories in a Caramel Macchiato. Round to two decimal places.
While many people count calories, some often don't think about calories in the beverages they consume. Starbucks, one of the leading coffeehouse chains, provides nutrition information about all of their beverages on their website. Nutrition information, including number of calories, fat (g), carbohydrates (g), and protein (g), was collected on a random sample of Starbucks' 16 ounce ("Grande") hot espresso drinks. Note that all of the drinks in the sample are made with 2% milk unless the name specifically included the term "Skinny,"
which is how Starbucks indicated a beverage made with nonfat milk.
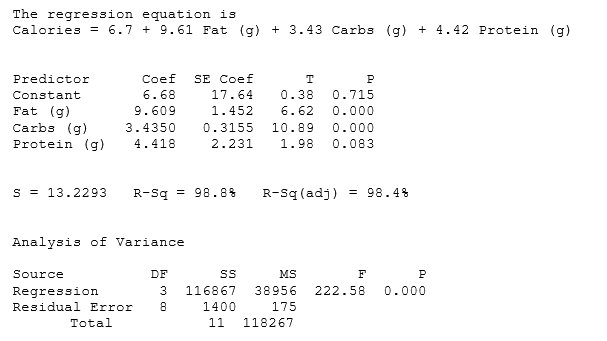
-The "Caramel Macchiato"
was one of the drinks selected for the sample. When made with 2% milk, a grande Caramel Macchiato has 7 grams of fat, 34 grams of carbohydrates, and 10 grams of protein. Predict the number of calories in a Caramel Macchiato. Round to two decimal places.
Unlock Deck
Unlock for access to all 72 flashcards in this deck.
Unlock Deck
k this deck
33
Use the following
While many people count calories, some often don't think about calories in the beverages they consume. Starbucks, one of the leading coffeehouse chains, provides nutrition information about all of their beverages on their website. Nutrition information, including number of calories, fat (g), carbohydrates (g), and protein (g), was collected on a random sample of Starbucks' 16 ounce ("Grande") hot espresso drinks. Note that all of the drinks in the sample are made with 2% milk unless the name specifically included the term "Skinny,"
which is how Starbucks indicated a beverage made with nonfat milk.
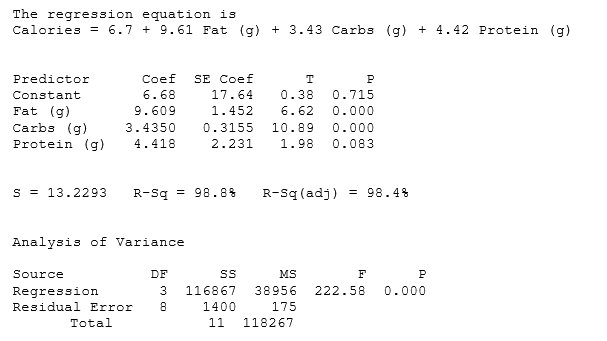
-Interpret the coefficient of Fat in context.
While many people count calories, some often don't think about calories in the beverages they consume. Starbucks, one of the leading coffeehouse chains, provides nutrition information about all of their beverages on their website. Nutrition information, including number of calories, fat (g), carbohydrates (g), and protein (g), was collected on a random sample of Starbucks' 16 ounce ("Grande") hot espresso drinks. Note that all of the drinks in the sample are made with 2% milk unless the name specifically included the term "Skinny,"
which is how Starbucks indicated a beverage made with nonfat milk.
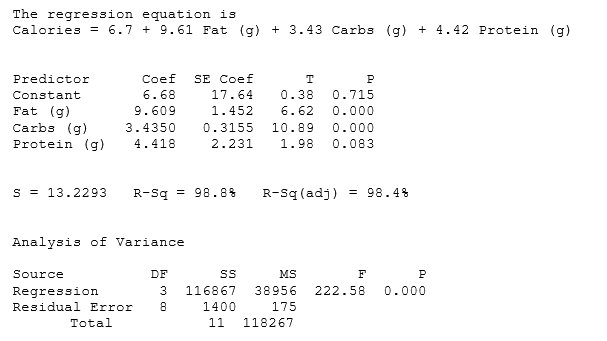
-Interpret the coefficient of Fat in context.
Unlock Deck
Unlock for access to all 72 flashcards in this deck.
Unlock Deck
k this deck
34
Use the following
While many people count calories, some often don't think about calories in the beverages they consume. Starbucks, one of the leading coffeehouse chains, provides nutrition information about all of their beverages on their website. Nutrition information, including number of calories, fat (g), carbohydrates (g), and protein (g), was collected on a random sample of Starbucks' 16 ounce ("Grande") hot espresso drinks. Note that all of the drinks in the sample are made with 2% milk unless the name specifically included the term "Skinny,"
which is how Starbucks indicated a beverage made with nonfat milk.
?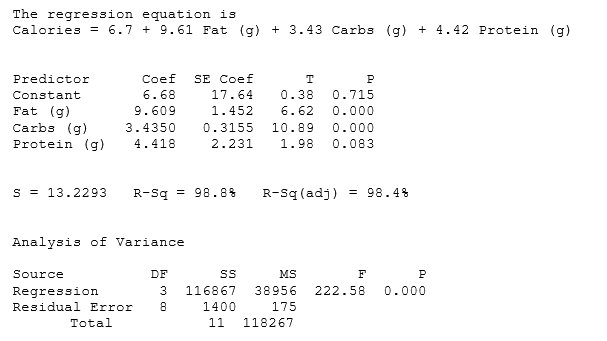
-How many drinks were used in this sample?
A) 12
B) 11
C) 10
D) 9
While many people count calories, some often don't think about calories in the beverages they consume. Starbucks, one of the leading coffeehouse chains, provides nutrition information about all of their beverages on their website. Nutrition information, including number of calories, fat (g), carbohydrates (g), and protein (g), was collected on a random sample of Starbucks' 16 ounce ("Grande") hot espresso drinks. Note that all of the drinks in the sample are made with 2% milk unless the name specifically included the term "Skinny,"
which is how Starbucks indicated a beverage made with nonfat milk.
?
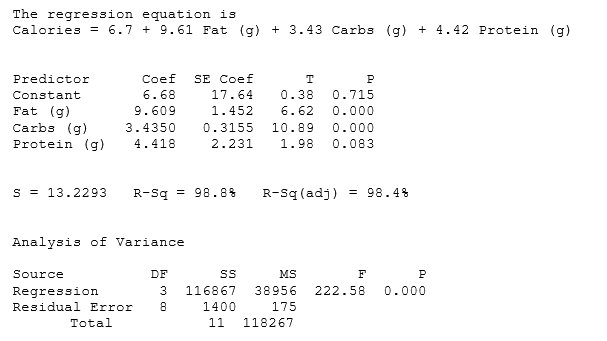
-How many drinks were used in this sample?
A) 12
B) 11
C) 10
D) 9
Unlock Deck
Unlock for access to all 72 flashcards in this deck.
Unlock Deck
k this deck
35
Use the following
While many people count calories, some often don't think about calories in the beverages they consume. Starbucks, one of the leading coffeehouse chains, provides nutrition information about all of their beverages on their website. Nutrition information, including number of calories, fat (g), carbohydrates (g), and protein (g), was collected on a random sample of Starbucks' 16 ounce ("Grande") hot espresso drinks. Note that all of the drinks in the sample are made with 2% milk unless the name specifically included the term "Skinny,"
which is how Starbucks indicated a beverage made with nonfat milk.
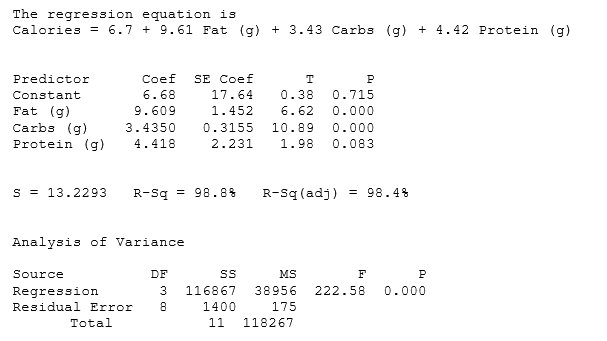
-Interpret R2 for this model.
While many people count calories, some often don't think about calories in the beverages they consume. Starbucks, one of the leading coffeehouse chains, provides nutrition information about all of their beverages on their website. Nutrition information, including number of calories, fat (g), carbohydrates (g), and protein (g), was collected on a random sample of Starbucks' 16 ounce ("Grande") hot espresso drinks. Note that all of the drinks in the sample are made with 2% milk unless the name specifically included the term "Skinny,"
which is how Starbucks indicated a beverage made with nonfat milk.
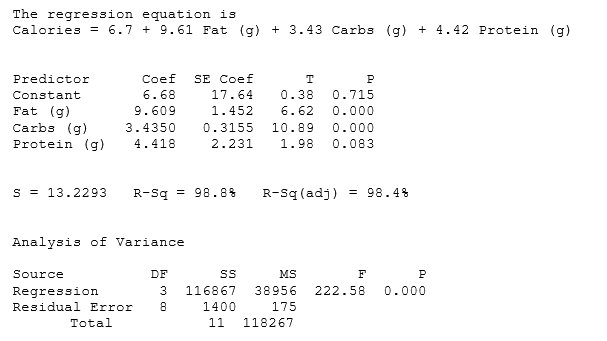
-Interpret R2 for this model.
Unlock Deck
Unlock for access to all 72 flashcards in this deck.
Unlock Deck
k this deck
36
Use the following
While many people count calories, some often don't think about calories in the beverages they consume. Starbucks, one of the leading coffeehouse chains, provides nutrition information about all of their beverages on their website. Nutrition information, including number of calories, fat (g), carbohydrates (g), and protein (g), was collected on a random sample of Starbucks' 16 ounce ("Grande") hot espresso drinks. Note that all of the drinks in the sample are made with 2% milk unless the name specifically included the term "Skinny,"
which is how Starbucks indicated a beverage made with nonfat milk.
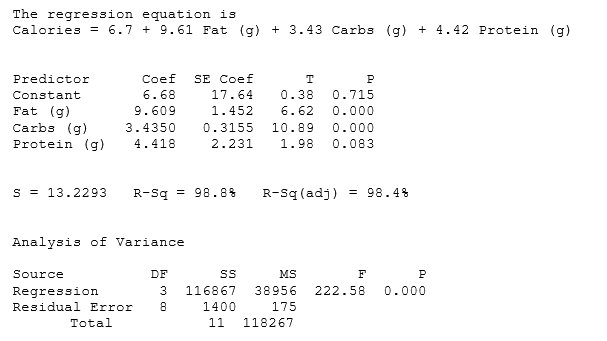
-Is the model effective according to the ANOVA test? Use a 5% significance level. Include all details of the test.
While many people count calories, some often don't think about calories in the beverages they consume. Starbucks, one of the leading coffeehouse chains, provides nutrition information about all of their beverages on their website. Nutrition information, including number of calories, fat (g), carbohydrates (g), and protein (g), was collected on a random sample of Starbucks' 16 ounce ("Grande") hot espresso drinks. Note that all of the drinks in the sample are made with 2% milk unless the name specifically included the term "Skinny,"
which is how Starbucks indicated a beverage made with nonfat milk.
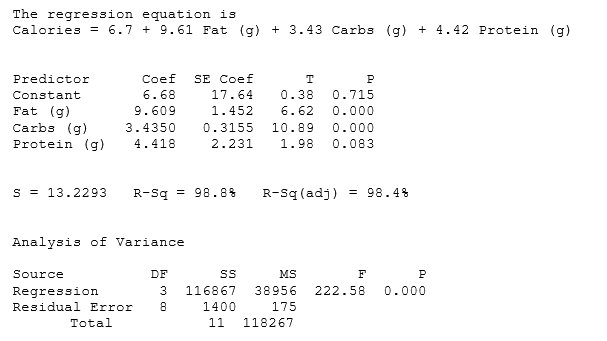
-Is the model effective according to the ANOVA test? Use a 5% significance level. Include all details of the test.
Unlock Deck
Unlock for access to all 72 flashcards in this deck.
Unlock Deck
k this deck
37
Use the following
While many people count calories, some often don't think about calories in the beverages they consume. Starbucks, one of the leading coffeehouse chains, provides nutrition information about all of their beverages on their website. Nutrition information, including number of calories, fat (g), carbohydrates (g), and protein (g), was collected on a random sample of Starbucks' 16 ounce ("Grande") hot espresso drinks. Note that all of the drinks in the sample are made with 2% milk unless the name specifically included the term "Skinny,"
which is how Starbucks indicated a beverage made with nonfat milk.
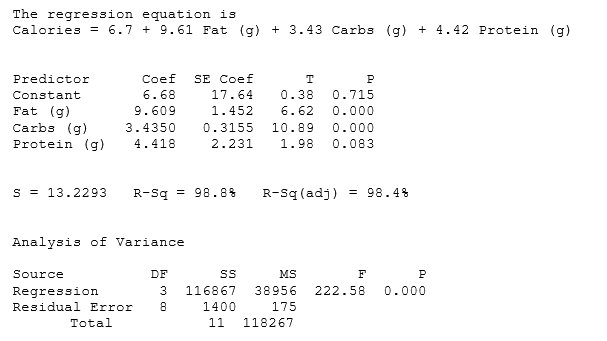
-Which predictors are significant at the 5% level? What are their p-values?
While many people count calories, some often don't think about calories in the beverages they consume. Starbucks, one of the leading coffeehouse chains, provides nutrition information about all of their beverages on their website. Nutrition information, including number of calories, fat (g), carbohydrates (g), and protein (g), was collected on a random sample of Starbucks' 16 ounce ("Grande") hot espresso drinks. Note that all of the drinks in the sample are made with 2% milk unless the name specifically included the term "Skinny,"
which is how Starbucks indicated a beverage made with nonfat milk.
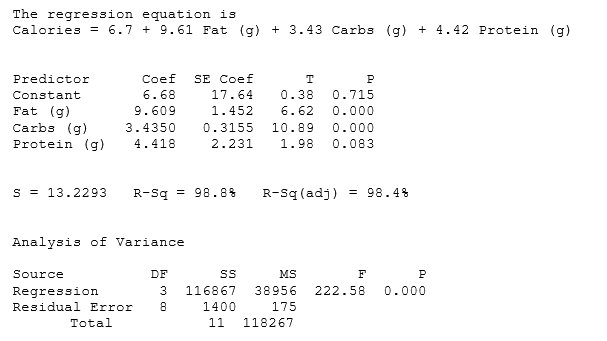
-Which predictors are significant at the 5% level? What are their p-values?
Unlock Deck
Unlock for access to all 72 flashcards in this deck.
Unlock Deck
k this deck
38
Use the following
While many people count calories, some often don't think about calories in the beverages they consume. Starbucks, one of the leading coffeehouse chains, provides nutrition information about all of their beverages on their website. Nutrition information, including number of calories, fat (g), carbohydrates (g), and protein (g), was collected on a random sample of Starbucks' 16 ounce ("Grande") hot espresso drinks. Note that all of the drinks in the sample are made with 2% milk unless the name specifically included the term "Skinny,"
which is how Starbucks indicated a beverage made with nonfat milk.
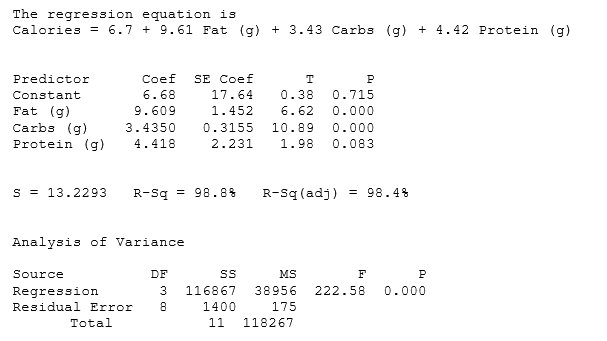
-A dotplot of the residuals and a scatterplot of the residuals versus the predicted values are provided. Discuss whether the conditions for a multiple linear regression are reasonable by referring to the appropriate plots.
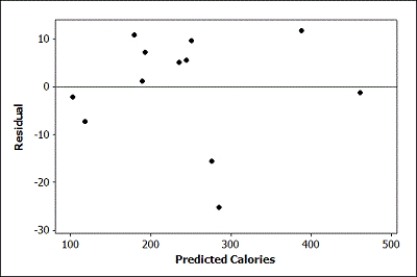
While many people count calories, some often don't think about calories in the beverages they consume. Starbucks, one of the leading coffeehouse chains, provides nutrition information about all of their beverages on their website. Nutrition information, including number of calories, fat (g), carbohydrates (g), and protein (g), was collected on a random sample of Starbucks' 16 ounce ("Grande") hot espresso drinks. Note that all of the drinks in the sample are made with 2% milk unless the name specifically included the term "Skinny,"
which is how Starbucks indicated a beverage made with nonfat milk.
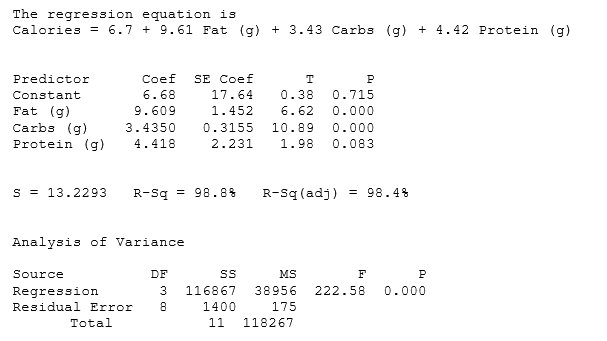
-A dotplot of the residuals and a scatterplot of the residuals versus the predicted values are provided. Discuss whether the conditions for a multiple linear regression are reasonable by referring to the appropriate plots.

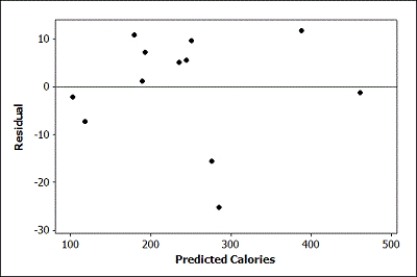
Unlock Deck
Unlock for access to all 72 flashcards in this deck.
Unlock Deck
k this deck
39
Use the following to answer questions :
A small university is concerned with monitoring the electricity usage in its Student Center, and its officials want to better understand what influences the amount of electricity used on a given day. They collected data on the amount of electricity used in the Student Center each day and the daily high temperature for nearly a year. They also made note of whether each day was a weekend or not (1 = Saturday/Sunday and 0 = Monday - Friday). Regression output is provided.
Helpful notes: 1) electricity usage is measured in kilowatt hours, 2) during the cold months the Student Center is heated by gas, not electricity, and 3) air conditioning the building during the warm months does use electricity.
The regression equation is Electricity = 83.6 + 0.529 High Temp - 25.2 Weekend
S = 29.8162 R-Sq = 24.7% R-Sq(adj) = 24.2%
Analysis of Variance

-Predict the amount of electricity used on a Monday with a high temperature of 62o F. Use one decimal place in your answer.
A small university is concerned with monitoring the electricity usage in its Student Center, and its officials want to better understand what influences the amount of electricity used on a given day. They collected data on the amount of electricity used in the Student Center each day and the daily high temperature for nearly a year. They also made note of whether each day was a weekend or not (1 = Saturday/Sunday and 0 = Monday - Friday). Regression output is provided.
Helpful notes: 1) electricity usage is measured in kilowatt hours, 2) during the cold months the Student Center is heated by gas, not electricity, and 3) air conditioning the building during the warm months does use electricity.
The regression equation is Electricity = 83.6 + 0.529 High Temp - 25.2 Weekend

S = 29.8162 R-Sq = 24.7% R-Sq(adj) = 24.2%
Analysis of Variance

-Predict the amount of electricity used on a Monday with a high temperature of 62o F. Use one decimal place in your answer.
Unlock Deck
Unlock for access to all 72 flashcards in this deck.
Unlock Deck
k this deck
40
Use the following to answer questions :
A small university is concerned with monitoring the electricity usage in its Student Center, and its officials want to better understand what influences the amount of electricity used on a given day. They collected data on the amount of electricity used in the Student Center each day and the daily high temperature for nearly a year. They also made note of whether each day was a weekend or not (1 = Saturday/Sunday and 0 = Monday - Friday). Regression output is provided.
Helpful notes: 1) electricity usage is measured in kilowatt hours, 2) during the cold months the Student Center is heated by gas, not electricity, and 3) air conditioning the building during the warm months does use electricity.
The regression equation is Electricity = 83.6 + 0.529 High Temp - 25.2 Weekend
S = 29.8162 R-Sq = 24.7% R-Sq(adj) = 24.2%
Analysis of Variance

-Predict the amount of electricity used on a Saturday with a high temperature of 68o F. Use one decimal place in your answer.
A small university is concerned with monitoring the electricity usage in its Student Center, and its officials want to better understand what influences the amount of electricity used on a given day. They collected data on the amount of electricity used in the Student Center each day and the daily high temperature for nearly a year. They also made note of whether each day was a weekend or not (1 = Saturday/Sunday and 0 = Monday - Friday). Regression output is provided.
Helpful notes: 1) electricity usage is measured in kilowatt hours, 2) during the cold months the Student Center is heated by gas, not electricity, and 3) air conditioning the building during the warm months does use electricity.
The regression equation is Electricity = 83.6 + 0.529 High Temp - 25.2 Weekend

S = 29.8162 R-Sq = 24.7% R-Sq(adj) = 24.2%
Analysis of Variance

-Predict the amount of electricity used on a Saturday with a high temperature of 68o F. Use one decimal place in your answer.
Unlock Deck
Unlock for access to all 72 flashcards in this deck.
Unlock Deck
k this deck
41
Use the following to answer questions :
A small university is concerned with monitoring the electricity usage in its Student Center, and its officials want to better understand what influences the amount of electricity used on a given day. They collected data on the amount of electricity used in the Student Center each day and the daily high temperature for nearly a year. They also made note of whether each day was a weekend or not (1 = Saturday/Sunday and 0 = Monday - Friday). Regression output is provided.
Helpful notes: 1) electricity usage is measured in kilowatt hours, 2) during the cold months the Student Center is heated by gas, not electricity, and 3) air conditioning the building during the warm months does use electricity.
The regression equation is Electricity = 83.6 + 0.529 High Temp - 25.2 Weekend
S = 29.8162 R-Sq = 24.7% R-Sq(adj) = 24.2%
Analysis of Variance

-Interpret the coefficient of High Temp in context.
A small university is concerned with monitoring the electricity usage in its Student Center, and its officials want to better understand what influences the amount of electricity used on a given day. They collected data on the amount of electricity used in the Student Center each day and the daily high temperature for nearly a year. They also made note of whether each day was a weekend or not (1 = Saturday/Sunday and 0 = Monday - Friday). Regression output is provided.
Helpful notes: 1) electricity usage is measured in kilowatt hours, 2) during the cold months the Student Center is heated by gas, not electricity, and 3) air conditioning the building during the warm months does use electricity.
The regression equation is Electricity = 83.6 + 0.529 High Temp - 25.2 Weekend

S = 29.8162 R-Sq = 24.7% R-Sq(adj) = 24.2%
Analysis of Variance

-Interpret the coefficient of High Temp in context.
Unlock Deck
Unlock for access to all 72 flashcards in this deck.
Unlock Deck
k this deck
42
Use the following to answer questions :
A small university is concerned with monitoring the electricity usage in its Student Center, and its officials want to better understand what influences the amount of electricity used on a given day. They collected data on the amount of electricity used in the Student Center each day and the daily high temperature for nearly a year. They also made note of whether each day was a weekend or not (1 = Saturday/Sunday and 0 = Monday - Friday). Regression output is provided.
Helpful notes: 1) electricity usage is measured in kilowatt hours, 2) during the cold months the Student Center is heated by gas, not electricity, and 3) air conditioning the building during the warm months does use electricity.
The regression equation is Electricity = 83.6 + 0.529 High Temp - 25.2 Weekend
S = 29.8162 R-Sq = 24.7% R-Sq(adj) = 24.2%
Analysis of Variance

-Interpret the coefficient of Weekend in context.
A small university is concerned with monitoring the electricity usage in its Student Center, and its officials want to better understand what influences the amount of electricity used on a given day. They collected data on the amount of electricity used in the Student Center each day and the daily high temperature for nearly a year. They also made note of whether each day was a weekend or not (1 = Saturday/Sunday and 0 = Monday - Friday). Regression output is provided.
Helpful notes: 1) electricity usage is measured in kilowatt hours, 2) during the cold months the Student Center is heated by gas, not electricity, and 3) air conditioning the building during the warm months does use electricity.
The regression equation is Electricity = 83.6 + 0.529 High Temp - 25.2 Weekend

S = 29.8162 R-Sq = 24.7% R-Sq(adj) = 24.2%
Analysis of Variance

-Interpret the coefficient of Weekend in context.
Unlock Deck
Unlock for access to all 72 flashcards in this deck.
Unlock Deck
k this deck
43
Use the following to answer questions :
A small university is concerned with monitoring the electricity usage in its Student Center, and its officials want to better understand what influences the amount of electricity used on a given day. They collected data on the amount of electricity used in the Student Center each day and the daily high temperature for nearly a year. They also made note of whether each day was a weekend or not (1 = Saturday/Sunday and 0 = Monday - Friday). Regression output is provided.
Helpful notes: 1) electricity usage is measured in kilowatt hours, 2) during the cold months the Student Center is heated by gas, not electricity, and 3) air conditioning the building during the warm months does use electricity.
The regression equation is Electricity = 83.6 + 0.529 High Temp - 25.2 Weekend
S = 29.8162 R-Sq = 24.7% R-Sq(adj) = 24.2%
Analysis of Variance

-How many days are included in the sample?
A) 365
B) 311
C) 312
D) 313
A small university is concerned with monitoring the electricity usage in its Student Center, and its officials want to better understand what influences the amount of electricity used on a given day. They collected data on the amount of electricity used in the Student Center each day and the daily high temperature for nearly a year. They also made note of whether each day was a weekend or not (1 = Saturday/Sunday and 0 = Monday - Friday). Regression output is provided.
Helpful notes: 1) electricity usage is measured in kilowatt hours, 2) during the cold months the Student Center is heated by gas, not electricity, and 3) air conditioning the building during the warm months does use electricity.
The regression equation is Electricity = 83.6 + 0.529 High Temp - 25.2 Weekend

S = 29.8162 R-Sq = 24.7% R-Sq(adj) = 24.2%
Analysis of Variance

-How many days are included in the sample?
A) 365
B) 311
C) 312
D) 313
Unlock Deck
Unlock for access to all 72 flashcards in this deck.
Unlock Deck
k this deck
44
Use the following to answer questions :
A small university is concerned with monitoring the electricity usage in its Student Center, and its officials want to better understand what influences the amount of electricity used on a given day. They collected data on the amount of electricity used in the Student Center each day and the daily high temperature for nearly a year. They also made note of whether each day was a weekend or not (1 = Saturday/Sunday and 0 = Monday - Friday). Regression output is provided.
Helpful notes: 1) electricity usage is measured in kilowatt hours, 2) during the cold months the Student Center is heated by gas, not electricity, and 3) air conditioning the building during the warm months does use electricity.
The regression equation is Electricity = 83.6 + 0.529 High Temp - 25.2 Weekend
S = 29.8162 R-Sq = 24.7% R-Sq(adj) = 24.2%
Analysis of Variance

-Interpret R2 for this model.
A small university is concerned with monitoring the electricity usage in its Student Center, and its officials want to better understand what influences the amount of electricity used on a given day. They collected data on the amount of electricity used in the Student Center each day and the daily high temperature for nearly a year. They also made note of whether each day was a weekend or not (1 = Saturday/Sunday and 0 = Monday - Friday). Regression output is provided.
Helpful notes: 1) electricity usage is measured in kilowatt hours, 2) during the cold months the Student Center is heated by gas, not electricity, and 3) air conditioning the building during the warm months does use electricity.
The regression equation is Electricity = 83.6 + 0.529 High Temp - 25.2 Weekend

S = 29.8162 R-Sq = 24.7% R-Sq(adj) = 24.2%
Analysis of Variance

-Interpret R2 for this model.
Unlock Deck
Unlock for access to all 72 flashcards in this deck.
Unlock Deck
k this deck
45
Use the following to answer questions :
A small university is concerned with monitoring the electricity usage in its Student Center, and its officials want to better understand what influences the amount of electricity used on a given day. They collected data on the amount of electricity used in the Student Center each day and the daily high temperature for nearly a year. They also made note of whether each day was a weekend or not (1 = Saturday/Sunday and 0 = Monday - Friday). Regression output is provided.
Helpful notes: 1) electricity usage is measured in kilowatt hours, 2) during the cold months the Student Center is heated by gas, not electricity, and 3) air conditioning the building during the warm months does use electricity.
The regression equation is Electricity = 83.6 + 0.529 High Temp - 25.2 Weekend
S = 29.8162 R-Sq = 24.7% R-Sq(adj) = 24.2%
Analysis of Variance

-Is the model effective according to the ANOVA test? Use =
0.05. Include all details of the test.
A small university is concerned with monitoring the electricity usage in its Student Center, and its officials want to better understand what influences the amount of electricity used on a given day. They collected data on the amount of electricity used in the Student Center each day and the daily high temperature for nearly a year. They also made note of whether each day was a weekend or not (1 = Saturday/Sunday and 0 = Monday - Friday). Regression output is provided.
Helpful notes: 1) electricity usage is measured in kilowatt hours, 2) during the cold months the Student Center is heated by gas, not electricity, and 3) air conditioning the building during the warm months does use electricity.
The regression equation is Electricity = 83.6 + 0.529 High Temp - 25.2 Weekend

S = 29.8162 R-Sq = 24.7% R-Sq(adj) = 24.2%
Analysis of Variance

-Is the model effective according to the ANOVA test? Use =

Unlock Deck
Unlock for access to all 72 flashcards in this deck.
Unlock Deck
k this deck
46
Use the following to answer questions :
A small university is concerned with monitoring the electricity usage in its Student Center, and its officials want to better understand what influences the amount of electricity used on a given day. They collected data on the amount of electricity used in the Student Center each day and the daily high temperature for nearly a year. They also made note of whether each day was a weekend or not (1 = Saturday/Sunday and 0 = Monday - Friday). Regression output is provided.
Helpful notes: 1) electricity usage is measured in kilowatt hours, 2) during the cold months the Student Center is heated by gas, not electricity, and 3) air conditioning the building during the warm months does use electricity.
The regression equation is Electricity = 83.6 + 0.529 High Temp - 25.2 Weekend
S = 29.8162 R-Sq = 24.7% R-Sq(adj) = 24.2%
Analysis of Variance

-Which predictors are significant at the 5% level? What are their p-values?
A small university is concerned with monitoring the electricity usage in its Student Center, and its officials want to better understand what influences the amount of electricity used on a given day. They collected data on the amount of electricity used in the Student Center each day and the daily high temperature for nearly a year. They also made note of whether each day was a weekend or not (1 = Saturday/Sunday and 0 = Monday - Friday). Regression output is provided.
Helpful notes: 1) electricity usage is measured in kilowatt hours, 2) during the cold months the Student Center is heated by gas, not electricity, and 3) air conditioning the building during the warm months does use electricity.
The regression equation is Electricity = 83.6 + 0.529 High Temp - 25.2 Weekend

S = 29.8162 R-Sq = 24.7% R-Sq(adj) = 24.2%
Analysis of Variance

-Which predictors are significant at the 5% level? What are their p-values?
Unlock Deck
Unlock for access to all 72 flashcards in this deck.
Unlock Deck
k this deck
47
Use the following to answer questions :
A small university is concerned with monitoring the electricity usage in its Student Center, and its officials want to better understand what influences the amount of electricity used on a given day. They collected data on the amount of electricity used in the Student Center each day and the daily high temperature for nearly a year. They also made note of whether each day was a weekend or not (1 = Saturday/Sunday and 0 = Monday - Friday). Regression output is provided.
Helpful notes: 1) electricity usage is measured in kilowatt hours, 2) during the cold months the Student Center is heated by gas, not electricity, and 3) air conditioning the building during the warm months does use electricity.
The regression equation is Electricity = 83.6 + 0.529 High Temp - 25.2 Weekend
S = 29.8162 R-Sq = 24.7% R-Sq(adj) = 24.2%
Analysis of Variance

-Another possible predictor they recorded was the average temperature over the course of each day. Regression output for the model that uses High Temp, Weekend, and Avg. Temp is provided. Explain why these results differ so drastically from those for the two-predictor model.
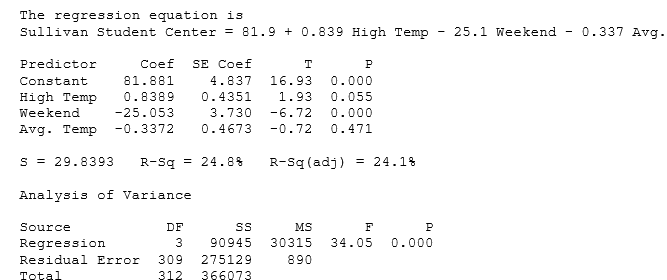
A small university is concerned with monitoring the electricity usage in its Student Center, and its officials want to better understand what influences the amount of electricity used on a given day. They collected data on the amount of electricity used in the Student Center each day and the daily high temperature for nearly a year. They also made note of whether each day was a weekend or not (1 = Saturday/Sunday and 0 = Monday - Friday). Regression output is provided.
Helpful notes: 1) electricity usage is measured in kilowatt hours, 2) during the cold months the Student Center is heated by gas, not electricity, and 3) air conditioning the building during the warm months does use electricity.
The regression equation is Electricity = 83.6 + 0.529 High Temp - 25.2 Weekend

S = 29.8162 R-Sq = 24.7% R-Sq(adj) = 24.2%
Analysis of Variance

-Another possible predictor they recorded was the average temperature over the course of each day. Regression output for the model that uses High Temp, Weekend, and Avg. Temp is provided. Explain why these results differ so drastically from those for the two-predictor model.
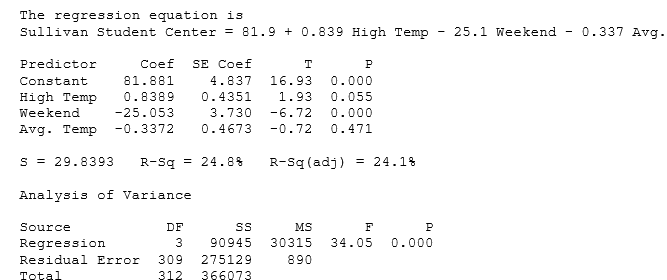
Unlock Deck
Unlock for access to all 72 flashcards in this deck.
Unlock Deck
k this deck
48
Use the following to answer questions :
A small university is concerned with monitoring the electricity usage in its Student Center, and its officials want to better understand what influences the amount of electricity used on a given day. They collected data on the amount of electricity used in the Student Center each day and the daily high temperature for nearly a year. They also made note of whether each day was a weekend or not (1 = Saturday/Sunday and 0 = Monday - Friday). Regression output is provided.
Helpful notes: 1) electricity usage is measured in kilowatt hours, 2) during the cold months the Student Center is heated by gas, not electricity, and 3) air conditioning the building during the warm months does use electricity.
The regression equation is Electricity = 83.6 + 0.529 High Temp - 25.2 Weekend
S = 29.8162 R-Sq = 24.7% R-Sq(adj) = 24.2%
Analysis of Variance

-A histogram of the residuals and a scatterplot of the residuals versus the predicted values are provided. Discuss whether the conditions for a multiple linear regression are reasonable by referring to the appropriate plots.
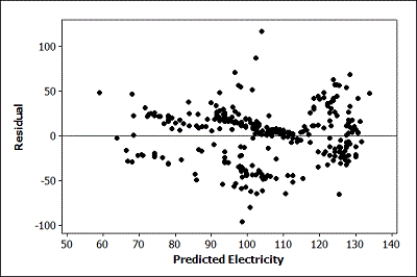
A small university is concerned with monitoring the electricity usage in its Student Center, and its officials want to better understand what influences the amount of electricity used on a given day. They collected data on the amount of electricity used in the Student Center each day and the daily high temperature for nearly a year. They also made note of whether each day was a weekend or not (1 = Saturday/Sunday and 0 = Monday - Friday). Regression output is provided.
Helpful notes: 1) electricity usage is measured in kilowatt hours, 2) during the cold months the Student Center is heated by gas, not electricity, and 3) air conditioning the building during the warm months does use electricity.
The regression equation is Electricity = 83.6 + 0.529 High Temp - 25.2 Weekend

S = 29.8162 R-Sq = 24.7% R-Sq(adj) = 24.2%
Analysis of Variance

-A histogram of the residuals and a scatterplot of the residuals versus the predicted values are provided. Discuss whether the conditions for a multiple linear regression are reasonable by referring to the appropriate plots.
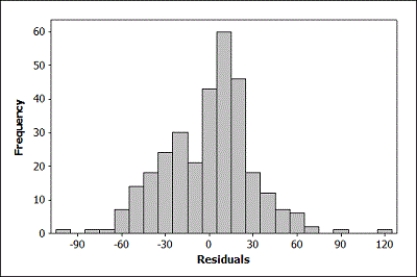
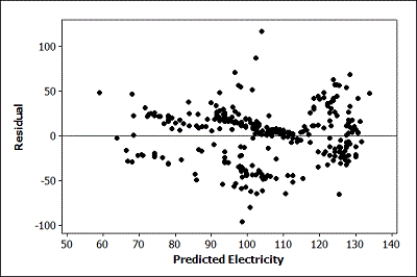
Unlock Deck
Unlock for access to all 72 flashcards in this deck.
Unlock Deck
k this deck
49
Use the following
Is there such thing as a "home court/field advantage"? Especially at the professional level? The number of points scored and whether or not it was a home game are available for a sample of games played by the Minnesota Timberwolves during the 2011-2012 regular season. The Home variable is coded as 1 = home game and 0 = away game.
The regression equation is Points Scored = 102 - 8.76 Home
S = 12.7430 R-Sq = 11.5% R-Sq(adj) = 6.6%
Analysis of Variance

-How many points are the Timberwolves predicted to score in a home game? Round to one decimal place.
Is there such thing as a "home court/field advantage"? Especially at the professional level? The number of points scored and whether or not it was a home game are available for a sample of games played by the Minnesota Timberwolves during the 2011-2012 regular season. The Home variable is coded as 1 = home game and 0 = away game.
The regression equation is Points Scored = 102 - 8.76 Home

S = 12.7430 R-Sq = 11.5% R-Sq(adj) = 6.6%
Analysis of Variance

-How many points are the Timberwolves predicted to score in a home game? Round to one decimal place.
Unlock Deck
Unlock for access to all 72 flashcards in this deck.
Unlock Deck
k this deck
50
Use the following
Is there such thing as a "home court/field advantage"? Especially at the professional level? The number of points scored and whether or not it was a home game are available for a sample of games played by the Minnesota Timberwolves during the 2011-2012 regular season. The Home variable is coded as 1 = home game and 0 = away game.
The regression equation is Points Scored = 102 - 8.76 Home
S = 12.7430 R-Sq = 11.5% R-Sq(adj) = 6.6%
Analysis of Variance

-How many points are the Timberwolves predicted to score in an away game? Round to one decimal place.
Is there such thing as a "home court/field advantage"? Especially at the professional level? The number of points scored and whether or not it was a home game are available for a sample of games played by the Minnesota Timberwolves during the 2011-2012 regular season. The Home variable is coded as 1 = home game and 0 = away game.
The regression equation is Points Scored = 102 - 8.76 Home

S = 12.7430 R-Sq = 11.5% R-Sq(adj) = 6.6%
Analysis of Variance

-How many points are the Timberwolves predicted to score in an away game? Round to one decimal place.
Unlock Deck
Unlock for access to all 72 flashcards in this deck.
Unlock Deck
k this deck
51
Use the following
Is there such thing as a "home court/field advantage"? Especially at the professional level? The number of points scored and whether or not it was a home game are available for a sample of games played by the Minnesota Timberwolves during the 2011-2012 regular season. The Home variable is coded as 1 = home game and 0 = away game.
The regression equation is Points Scored = 102 - 8.76 Home
S = 12.7430 R-Sq = 11.5% R-Sq(adj) = 6.6%
Analysis of Variance

-Interpret the R2 for this model.
Is there such thing as a "home court/field advantage"? Especially at the professional level? The number of points scored and whether or not it was a home game are available for a sample of games played by the Minnesota Timberwolves during the 2011-2012 regular season. The Home variable is coded as 1 = home game and 0 = away game.
The regression equation is Points Scored = 102 - 8.76 Home

S = 12.7430 R-Sq = 11.5% R-Sq(adj) = 6.6%
Analysis of Variance

-Interpret the R2 for this model.
Unlock Deck
Unlock for access to all 72 flashcards in this deck.
Unlock Deck
k this deck
52
Use the following
Is there such thing as a "home court/field advantage"? Especially at the professional level? The number of points scored and whether or not it was a home game are available for a sample of games played by the Minnesota Timberwolves during the 2011-2012 regular season. The Home variable is coded as 1 = home game and 0 = away game.
The regression equation is Points Scored = 102 - 8.76 Home
S = 12.7430 R-Sq = 11.5% R-Sq(adj) = 6.6%
Analysis of Variance

-Using
= 0.05, is there a difference in the number of points scored for home and away games? Include all details of the test.
Is there such thing as a "home court/field advantage"? Especially at the professional level? The number of points scored and whether or not it was a home game are available for a sample of games played by the Minnesota Timberwolves during the 2011-2012 regular season. The Home variable is coded as 1 = home game and 0 = away game.
The regression equation is Points Scored = 102 - 8.76 Home

S = 12.7430 R-Sq = 11.5% R-Sq(adj) = 6.6%
Analysis of Variance

-Using

Unlock Deck
Unlock for access to all 72 flashcards in this deck.
Unlock Deck
k this deck
53
Use the following
Does the price of used cars depend upon the model? Data were collected on the selling price and age of used Hyundai Elantras (coded as Model = 1) and Toyota Camrys (coded as Model = 0). Output from the multiple regression analysis is provided.
The regression equation is Price = 14.5 - 0.619 Age - 3.63 Model
S = 2.63465 R-Sq = 69.3% R-Sq(adj) = 68.4%
Analysis of Variance

-What is the predicted price of a 6-year-old Hyundai Elantra? Round to three decimal places.
Does the price of used cars depend upon the model? Data were collected on the selling price and age of used Hyundai Elantras (coded as Model = 1) and Toyota Camrys (coded as Model = 0). Output from the multiple regression analysis is provided.
The regression equation is Price = 14.5 - 0.619 Age - 3.63 Model

S = 2.63465 R-Sq = 69.3% R-Sq(adj) = 68.4%
Analysis of Variance

-What is the predicted price of a 6-year-old Hyundai Elantra? Round to three decimal places.
Unlock Deck
Unlock for access to all 72 flashcards in this deck.
Unlock Deck
k this deck
54
Use the following
Does the price of used cars depend upon the model? Data were collected on the selling price and age of used Hyundai Elantras (coded as Model = 1) and Toyota Camrys (coded as Model = 0). Output from the multiple regression analysis is provided.
The regression equation is Price = 14.5 - 0.619 Age - 3.63 Model
S = 2.63465 R-Sq = 69.3% R-Sq(adj) = 68.4%
Analysis of Variance

-What is the predicted price of a 6-year-old Toyota Camry? Round to three decimal places.
Does the price of used cars depend upon the model? Data were collected on the selling price and age of used Hyundai Elantras (coded as Model = 1) and Toyota Camrys (coded as Model = 0). Output from the multiple regression analysis is provided.
The regression equation is Price = 14.5 - 0.619 Age - 3.63 Model

S = 2.63465 R-Sq = 69.3% R-Sq(adj) = 68.4%
Analysis of Variance

-What is the predicted price of a 6-year-old Toyota Camry? Round to three decimal places.
Unlock Deck
Unlock for access to all 72 flashcards in this deck.
Unlock Deck
k this deck
55
Use the following
Does the price of used cars depend upon the model? Data were collected on the selling price and age of used Hyundai Elantras (coded as Model = 1) and Toyota Camrys (coded as Model = 0). Output from the multiple regression analysis is provided.
The regression equation is Price = 14.5 - 0.619 Age - 3.63 Model
S = 2.63465 R-Sq = 69.3% R-Sq(adj) = 68.4%
Analysis of Variance

-Interpret the coefficient of Model in context.
Does the price of used cars depend upon the model? Data were collected on the selling price and age of used Hyundai Elantras (coded as Model = 1) and Toyota Camrys (coded as Model = 0). Output from the multiple regression analysis is provided.
The regression equation is Price = 14.5 - 0.619 Age - 3.63 Model

S = 2.63465 R-Sq = 69.3% R-Sq(adj) = 68.4%
Analysis of Variance

-Interpret the coefficient of Model in context.
Unlock Deck
Unlock for access to all 72 flashcards in this deck.
Unlock Deck
k this deck
56
Use the following
Does the price of used cars depend upon the model? Data were collected on the selling price and age of used Hyundai Elantras (coded as Model = 1) and Toyota Camrys (coded as Model = 0). Output from the multiple regression analysis is provided.
The regression equation is Price = 14.5 - 0.619 Age - 3.63 Model
S = 2.63465 R-Sq = 69.3% R-Sq(adj) = 68.4%
Analysis of Variance

-Interpret R2 for this model.
Does the price of used cars depend upon the model? Data were collected on the selling price and age of used Hyundai Elantras (coded as Model = 1) and Toyota Camrys (coded as Model = 0). Output from the multiple regression analysis is provided.
The regression equation is Price = 14.5 - 0.619 Age - 3.63 Model

S = 2.63465 R-Sq = 69.3% R-Sq(adj) = 68.4%
Analysis of Variance

-Interpret R2 for this model.
Unlock Deck
Unlock for access to all 72 flashcards in this deck.
Unlock Deck
k this deck
57
Use the following
Does the price of used cars depend upon the model? Data were collected on the selling price and age of used Hyundai Elantras (coded as Model = 1) and Toyota Camrys (coded as Model = 0). Output from the multiple regression analysis is provided.
The regression equation is Price = 14.5 - 0.619 Age - 3.63 Model
S = 2.63465 R-Sq = 69.3% R-Sq(adj) = 68.4%
Analysis of Variance

-Is the model effective according to the ANOVA test? Use
= 0.05. Include all details of the test.
Does the price of used cars depend upon the model? Data were collected on the selling price and age of used Hyundai Elantras (coded as Model = 1) and Toyota Camrys (coded as Model = 0). Output from the multiple regression analysis is provided.
The regression equation is Price = 14.5 - 0.619 Age - 3.63 Model

S = 2.63465 R-Sq = 69.3% R-Sq(adj) = 68.4%
Analysis of Variance

-Is the model effective according to the ANOVA test? Use

Unlock Deck
Unlock for access to all 72 flashcards in this deck.
Unlock Deck
k this deck
58
Use the following
Does the price of used cars depend upon the model? Data were collected on the selling price and age of used Hyundai Elantras (coded as Model = 1) and Toyota Camrys (coded as Model = 0). Output from the multiple regression analysis is provided.
The regression equation is Price = 14.5 - 0.619 Age - 3.63 Model
S = 2.63465 R-Sq = 69.3% R-Sq(adj) = 68.4%
Analysis of Variance

-Which predictors are significant at the 5% level? What are their p-values?
Does the price of used cars depend upon the model? Data were collected on the selling price and age of used Hyundai Elantras (coded as Model = 1) and Toyota Camrys (coded as Model = 0). Output from the multiple regression analysis is provided.
The regression equation is Price = 14.5 - 0.619 Age - 3.63 Model

S = 2.63465 R-Sq = 69.3% R-Sq(adj) = 68.4%
Analysis of Variance

-Which predictors are significant at the 5% level? What are their p-values?
Unlock Deck
Unlock for access to all 72 flashcards in this deck.
Unlock Deck
k this deck
59
Use the following
Does the price of used cars depend upon the model? Data were collected on the selling price and age of used Hyundai Elantras (coded as Model = 1) and Toyota Camrys (coded as Model = 0). Output from the multiple regression analysis is provided.
The regression equation is Price = 14.5 - 0.619 Age - 3.63 Model
S = 2.63465 R-Sq = 69.3% R-Sq(adj) = 68.4%
Analysis of Variance

-A histogram of the residuals and a scatterplot of the residuals versus the predicted values are provided. Discuss whether the conditions for a multiple linear regression are reasonable by referring to the appropriate plots.
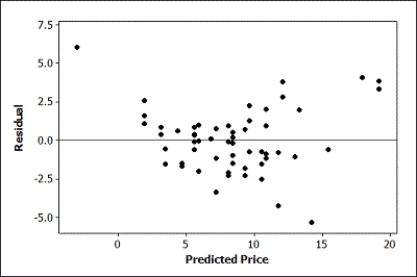
Does the price of used cars depend upon the model? Data were collected on the selling price and age of used Hyundai Elantras (coded as Model = 1) and Toyota Camrys (coded as Model = 0). Output from the multiple regression analysis is provided.
The regression equation is Price = 14.5 - 0.619 Age - 3.63 Model

S = 2.63465 R-Sq = 69.3% R-Sq(adj) = 68.4%
Analysis of Variance

-A histogram of the residuals and a scatterplot of the residuals versus the predicted values are provided. Discuss whether the conditions for a multiple linear regression are reasonable by referring to the appropriate plots.
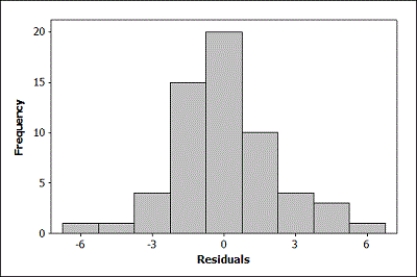
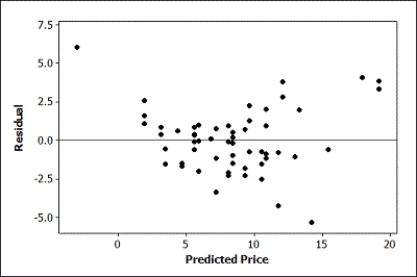
Unlock Deck
Unlock for access to all 72 flashcards in this deck.
Unlock Deck
k this deck
60
Use the following
Does the price of used cars depend upon the model? Data were collected on the selling price and age of used Hyundai Elantras (coded as Model = 1) and Toyota Camrys (coded as Model = 0). Output from the multiple regression analysis is provided.
The regression equation is Price = 14.5 - 0.619 Age - 3.63 Model
S = 2.63465 R-Sq = 69.3% R-Sq(adj) = 68.4%
Analysis of Variance

-Which of the following scatterplots of the residuals versus the predicted values does not indicate problems with either the linearity or the consistent variability conditions?
A)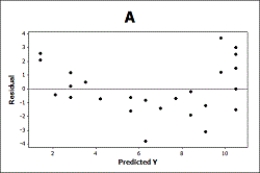
B)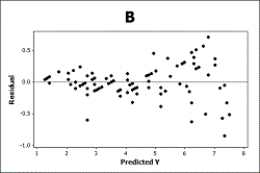
C)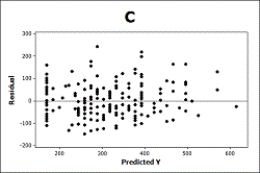
Does the price of used cars depend upon the model? Data were collected on the selling price and age of used Hyundai Elantras (coded as Model = 1) and Toyota Camrys (coded as Model = 0). Output from the multiple regression analysis is provided.
The regression equation is Price = 14.5 - 0.619 Age - 3.63 Model

S = 2.63465 R-Sq = 69.3% R-Sq(adj) = 68.4%
Analysis of Variance

-Which of the following scatterplots of the residuals versus the predicted values does not indicate problems with either the linearity or the consistent variability conditions?
A)
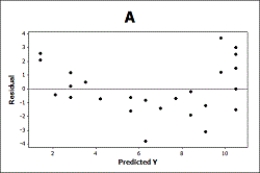
B)
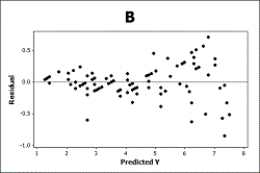
C)
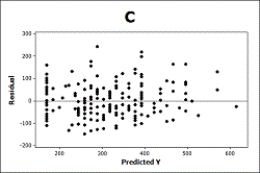
Unlock Deck
Unlock for access to all 72 flashcards in this deck.
Unlock Deck
k this deck
61
Use the following
The ANOVA table from a multiple regression analysis is provided.

-How many predictors are in the model?
The ANOVA table from a multiple regression analysis is provided.

-How many predictors are in the model?
Unlock Deck
Unlock for access to all 72 flashcards in this deck.
Unlock Deck
k this deck
62
Use the following
The ANOVA table from a multiple regression analysis is provided.

-How large is the sample size?
The ANOVA table from a multiple regression analysis is provided.

-How large is the sample size?
Unlock Deck
Unlock for access to all 72 flashcards in this deck.
Unlock Deck
k this deck
63
Use the following
The ANOVA table from a multiple regression analysis is provided.

-Compute R2 for this model. Round to three decimal places.
The ANOVA table from a multiple regression analysis is provided.

-Compute R2 for this model. Round to three decimal places.
Unlock Deck
Unlock for access to all 72 flashcards in this deck.
Unlock Deck
k this deck
64
Use the following
Output for a model to predict the GPAs of students at a small university based on their Math SAT scores, Verbal SAT scores, and the number of hours spent watching television in a typical week is provided.
The regression equation is
GPA = 1.80 + 0.00104 Math SAT + 0.00142 Verbal SAT - 0.0147 TV
S = 0.366780 R-Sq = ?% R-Sq(adj) = 19.0%
Analysis of Variance

-Predict the GPA of a student at this university with a Math SAT score of 600, a Verbal SAT score of 580, and who watches 5 hours of television in a typical week. Round to three decimal places.
Output for a model to predict the GPAs of students at a small university based on their Math SAT scores, Verbal SAT scores, and the number of hours spent watching television in a typical week is provided.
The regression equation is
GPA = 1.80 + 0.00104 Math SAT + 0.00142 Verbal SAT - 0.0147 TV

S = 0.366780 R-Sq = ?% R-Sq(adj) = 19.0%
Analysis of Variance

-Predict the GPA of a student at this university with a Math SAT score of 600, a Verbal SAT score of 580, and who watches 5 hours of television in a typical week. Round to three decimal places.
Unlock Deck
Unlock for access to all 72 flashcards in this deck.
Unlock Deck
k this deck
65
Use the following
Output for a model to predict the GPAs of students at a small university based on their Math SAT scores, Verbal SAT scores, and the number of hours spent watching television in a typical week is provided.
The regression equation is
GPA = 1.80 + 0.00104 Math SAT + 0.00142 Verbal SAT - 0.0147 TV
S = 0.366780 R-Sq = ?% R-Sq(adj) = 19.0%
Analysis of Variance

-Interpret the coefficient of TV in context.
Output for a model to predict the GPAs of students at a small university based on their Math SAT scores, Verbal SAT scores, and the number of hours spent watching television in a typical week is provided.
The regression equation is
GPA = 1.80 + 0.00104 Math SAT + 0.00142 Verbal SAT - 0.0147 TV

S = 0.366780 R-Sq = ?% R-Sq(adj) = 19.0%
Analysis of Variance

-Interpret the coefficient of TV in context.
Unlock Deck
Unlock for access to all 72 flashcards in this deck.
Unlock Deck
k this deck
66
Use the following
Output for a model to predict the GPAs of students at a small university based on their Math SAT scores, Verbal SAT scores, and the number of hours spent watching television in a typical week is provided.
The regression equation is
GPA = 1.80 + 0.00104 Math SAT + 0.00142 Verbal SAT - 0.0147 TV
S = 0.366780 R-Sq = ?% R-Sq(adj) = 19.0%
Analysis of Variance

-The R2 for this model is missing in the provided output. Use the available information to compute (round to three decimal places) and interpret R2 for this model.
Output for a model to predict the GPAs of students at a small university based on their Math SAT scores, Verbal SAT scores, and the number of hours spent watching television in a typical week is provided.
The regression equation is
GPA = 1.80 + 0.00104 Math SAT + 0.00142 Verbal SAT - 0.0147 TV

S = 0.366780 R-Sq = ?% R-Sq(adj) = 19.0%
Analysis of Variance

-The R2 for this model is missing in the provided output. Use the available information to compute (round to three decimal places) and interpret R2 for this model.
Unlock Deck
Unlock for access to all 72 flashcards in this deck.
Unlock Deck
k this deck
67
Use the following
Output for a model to predict the GPAs of students at a small university based on their Math SAT scores, Verbal SAT scores, and the number of hours spent watching television in a typical week is provided.
The regression equation is
GPA = 1.80 + 0.00104 Math SAT + 0.00142 Verbal SAT - 0.0147 TV
S = 0.366780 R-Sq = ?% R-Sq(adj) = 19.0%
Analysis of Variance

-Use the output to determine how many students were included in the sample.
Output for a model to predict the GPAs of students at a small university based on their Math SAT scores, Verbal SAT scores, and the number of hours spent watching television in a typical week is provided.
The regression equation is
GPA = 1.80 + 0.00104 Math SAT + 0.00142 Verbal SAT - 0.0147 TV

S = 0.366780 R-Sq = ?% R-Sq(adj) = 19.0%
Analysis of Variance

-Use the output to determine how many students were included in the sample.
Unlock Deck
Unlock for access to all 72 flashcards in this deck.
Unlock Deck
k this deck
68
Use the following
Output for a model to predict the GPAs of students at a small university based on their Math SAT scores, Verbal SAT scores, and the number of hours spent watching television in a typical week is provided.
The regression equation is
GPA = 1.80 + 0.00104 Math SAT + 0.00142 Verbal SAT - 0.0147 TV
S = 0.366780 R-Sq = ?% R-Sq(adj) = 19.0%
Analysis of Variance

-Some of the information in the ANOVA table is missing. How many degrees of freedom should appear in the "Regression"
row of the table?
Output for a model to predict the GPAs of students at a small university based on their Math SAT scores, Verbal SAT scores, and the number of hours spent watching television in a typical week is provided.
The regression equation is
GPA = 1.80 + 0.00104 Math SAT + 0.00142 Verbal SAT - 0.0147 TV

S = 0.366780 R-Sq = ?% R-Sq(adj) = 19.0%
Analysis of Variance

-Some of the information in the ANOVA table is missing. How many degrees of freedom should appear in the "Regression"
row of the table?
Unlock Deck
Unlock for access to all 72 flashcards in this deck.
Unlock Deck
k this deck
69
Use the following
Output for a model to predict the GPAs of students at a small university based on their Math SAT scores, Verbal SAT scores, and the number of hours spent watching television in a typical week is provided.
The regression equation is
GPA = 1.80 + 0.00104 Math SAT + 0.00142 Verbal SAT - 0.0147 TV
S = 0.366780 R-Sq = ?% R-Sq(adj) = 19.0%
Analysis of Variance

-Some of the information in the ANOVA table is missing. How many degrees of freedom should be listed in the "Residual Error"
row?
Output for a model to predict the GPAs of students at a small university based on their Math SAT scores, Verbal SAT scores, and the number of hours spent watching television in a typical week is provided.
The regression equation is
GPA = 1.80 + 0.00104 Math SAT + 0.00142 Verbal SAT - 0.0147 TV

S = 0.366780 R-Sq = ?% R-Sq(adj) = 19.0%
Analysis of Variance

-Some of the information in the ANOVA table is missing. How many degrees of freedom should be listed in the "Residual Error"
row?
Unlock Deck
Unlock for access to all 72 flashcards in this deck.
Unlock Deck
k this deck
70
Use the following
Output for a model to predict the GPAs of students at a small university based on their Math SAT scores, Verbal SAT scores, and the number of hours spent watching television in a typical week is provided.
The regression equation is
GPA = 1.80 + 0.00104 Math SAT + 0.00142 Verbal SAT - 0.0147 TV
S = 0.366780 R-Sq = ?% R-Sq(adj) = 19.0%
Analysis of Variance

-At the 5% significance level, is the model effective according to the ANOVA test. Include all details of the test.
Output for a model to predict the GPAs of students at a small university based on their Math SAT scores, Verbal SAT scores, and the number of hours spent watching television in a typical week is provided.
The regression equation is
GPA = 1.80 + 0.00104 Math SAT + 0.00142 Verbal SAT - 0.0147 TV

S = 0.366780 R-Sq = ?% R-Sq(adj) = 19.0%
Analysis of Variance

-At the 5% significance level, is the model effective according to the ANOVA test. Include all details of the test.
Unlock Deck
Unlock for access to all 72 flashcards in this deck.
Unlock Deck
k this deck
71
Use the following
Output for a model to predict the GPAs of students at a small university based on their Math SAT scores, Verbal SAT scores, and the number of hours spent watching television in a typical week is provided.
The regression equation is
GPA = 1.80 + 0.00104 Math SAT + 0.00142 Verbal SAT - 0.0147 TV
S = 0.366780 R-Sq = ?% R-Sq(adj) = 19.0%
Analysis of Variance

-Which predictors are significant at the 5% level? What are their p-values?
Output for a model to predict the GPAs of students at a small university based on their Math SAT scores, Verbal SAT scores, and the number of hours spent watching television in a typical week is provided.
The regression equation is
GPA = 1.80 + 0.00104 Math SAT + 0.00142 Verbal SAT - 0.0147 TV

S = 0.366780 R-Sq = ?% R-Sq(adj) = 19.0%
Analysis of Variance

-Which predictors are significant at the 5% level? What are their p-values?
Unlock Deck
Unlock for access to all 72 flashcards in this deck.
Unlock Deck
k this deck
72
Use the following
Output for a model to predict the GPAs of students at a small university based on their Math SAT scores, Verbal SAT scores, and the number of hours spent watching television in a typical week is provided.
The regression equation is
GPA = 1.80 + 0.00104 Math SAT + 0.00142 Verbal SAT - 0.0147 TV
S = 0.366780 R-Sq = ?% R-Sq(adj) = 19.0%
Analysis of Variance

-A dotplot of the residuals and a scatterplot of the residuals versus the predicted values are provided. Discuss whether the conditions for a multiple linear regression are reasonable by referring to the appropriate plots.
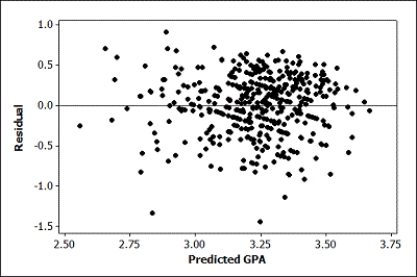
Output for a model to predict the GPAs of students at a small university based on their Math SAT scores, Verbal SAT scores, and the number of hours spent watching television in a typical week is provided.
The regression equation is
GPA = 1.80 + 0.00104 Math SAT + 0.00142 Verbal SAT - 0.0147 TV

S = 0.366780 R-Sq = ?% R-Sq(adj) = 19.0%
Analysis of Variance

-A dotplot of the residuals and a scatterplot of the residuals versus the predicted values are provided. Discuss whether the conditions for a multiple linear regression are reasonable by referring to the appropriate plots.
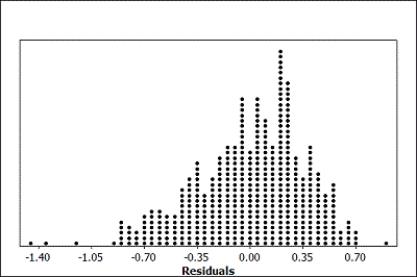
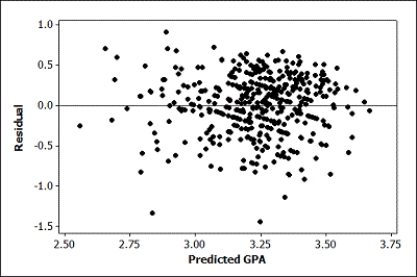
Unlock Deck
Unlock for access to all 72 flashcards in this deck.
Unlock Deck
k this deck